Colorectal cancer digital pathological image discrimination method and system based on weak supervised learning
A technology for digital pathology and colorectal cancer, applied in the field of pathological images, can solve problems such as difficult data labeling tasks, wrong labeling, and time-consuming
- Summary
- Abstract
- Description
- Claims
- Application Information
AI Technical Summary
Problems solved by technology
Method used
Image
Examples
Embodiment 1
[0065] The colorectal cancer digital pathology image discrimination system based on weakly supervised learning provided in this embodiment, such as Figure 1-2 shown, including:
[0066] The collection module 11 is used to collect digital pathological image data sets of colorectal cancer;
[0067] The preprocessing module 12 is used to preprocess the data in the collected data set to obtain preprocessed data;
[0068] The first classification module 13 is used to construct a sampling block discrimination model based on a weakly supervised learning algorithm, and input the preprocessed data into the constructed sampling block discrimination model for processing to obtain the classification results of all pathological image blocks in the full-slice sampling package ;
[0069] The second classification module 14 is configured to construct a decision fusion model, and input the obtained classification results of pathological image blocks into the decision fusion model for fusion...
Embodiment 2
[0194] The difference between the colorectal cancer digital pathology image discrimination system based on weakly supervised learning provided in this embodiment and the first embodiment is that:
[0195] In this embodiment, the performance of the algorithm is tested on the lesion-assisted diagnosis of colorectal cancer and the identification of microsatellite instability.
[0196] The experimental configuration is shown in Table 2:
[0197]
[0198] Table 2 Experimental hardware environment configuration
[0199] results and analysis:
[0200] (1) Auxiliary diagnosis of colorectal cancer lesions
[0201] In the lesion-assisted diagnosis task, the colorectal cancer pathology segmentation challenge data from MICCAI is used. The data contains 410 positive samples and 250 negative samples, and all positive samples are marked by pathologists for the lesion area. In this experiment, the purpose is to explore the multi-instance learning algorithm based on coarse-grained annot...
Embodiment 3
[0224] This embodiment provides a colorectal cancer digital pathology image discrimination method based on weakly supervised learning, including:
[0225] S1. Acquisition of colorectal cancer digital pathology image data set;
[0226] S2. Preprocessing the data in the collected data set to obtain preprocessed data;
[0227] S3. Construct a sampling block discrimination model based on a weakly supervised learning algorithm, and input the preprocessed data into the constructed sampling block discrimination model for processing, and obtain the classification results of all pathological image blocks in the full-slice sampling package;
[0228] S4. Build a decision-making fusion model, and input the obtained classification results of the pathological image blocks into the decision-making fusion model for fusion processing to obtain the classification results of the full-digital pathological images.
[0229] Further, the step S2 includes:
[0230] S21. Locate valid tissues in the ...
PUM
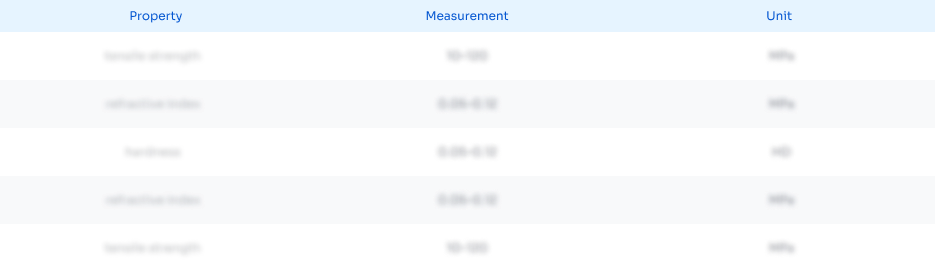
Abstract
Description
Claims
Application Information
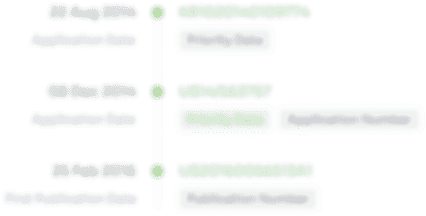
- R&D Engineer
- R&D Manager
- IP Professional
- Industry Leading Data Capabilities
- Powerful AI technology
- Patent DNA Extraction
Browse by: Latest US Patents, China's latest patents, Technical Efficacy Thesaurus, Application Domain, Technology Topic, Popular Technical Reports.
© 2024 PatSnap. All rights reserved.Legal|Privacy policy|Modern Slavery Act Transparency Statement|Sitemap|About US| Contact US: help@patsnap.com