Electrocardio diagnosis model and electrocardio detection device
A technology of diagnosis model and detection device, applied in the directions of diagnosis recording/measurement, diagnosis, medical science, etc., can solve the problems of wrong judgment, large difference, weight and coefficient limitation, etc., and achieve the effect of reducing error
- Summary
- Abstract
- Description
- Claims
- Application Information
AI Technical Summary
Problems solved by technology
Method used
Image
Examples
Embodiment
[0030] The present embodiment provides a kind of electrocardiogram diagnosis model electrocardiogram diagnosis model, comprises the following steps:
[0031] S1, collecting N 12-lead resting ECG data, the number of atrial fibrillation ECG data and non-atrial fibrillation ECG data is equal;
[0032] S2, perform preprocessing: if the signal sampling frequency is lower than 200Hz, first resample to make the sampling frequency above 200Hz, and then use a filter to filter;
[0033] S3, training deep learning network: the deep learning network includes at least 3 convolutional layers as feature extraction modules, and at least 2 fully connected layers as classification modules;
[0034] S4, optimize the parameters of the deep learning network by minimizing the loss value of the loss function, and minimize the loss value by using the proximal gradient descent method to obtain all the weights and offsets in the entire deep learning network.
[0035] In the signal optimization method ...
PUM
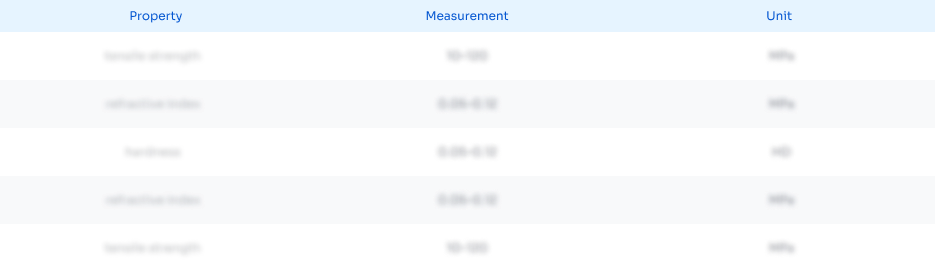
Abstract
Description
Claims
Application Information
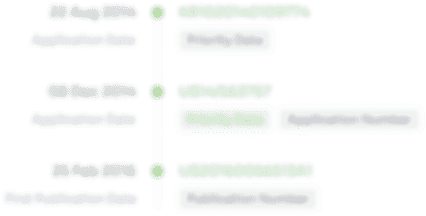
- R&D Engineer
- R&D Manager
- IP Professional
- Industry Leading Data Capabilities
- Powerful AI technology
- Patent DNA Extraction
Browse by: Latest US Patents, China's latest patents, Technical Efficacy Thesaurus, Application Domain, Technology Topic, Popular Technical Reports.
© 2024 PatSnap. All rights reserved.Legal|Privacy policy|Modern Slavery Act Transparency Statement|Sitemap|About US| Contact US: help@patsnap.com