Safety helmet wearing inspection method based on YOLOv3 algorithm
An inspection method and helmet technology, applied in neural learning methods, computing, computer parts, etc., can solve the problems of insufficient detection accuracy, inaccurate detection results, and difficulty in image feature extraction, so as to improve efficiency and accuracy, and improve iteration. The effect of computational efficiency
- Summary
- Abstract
- Description
- Claims
- Application Information
AI Technical Summary
Problems solved by technology
Method used
Image
Examples
Embodiment 1
[0051] A kind of safety helmet wearing inspection method based on YOLOv3 algorithm of the present embodiment, such as figure 1 As shown, the specific process steps are as follows:
[0052] Before labeling the sample pictures, it is necessary to construct a sample image set. The sample image set is made by collecting pictures of workshop staff wearing hard hats, pictures of staff not wearing hard hats, and scene pictures without human bodies. The sample image set The collection steps are as follows:
[0053] Step a. Collect the video of the staff wearing safety helmets in the workshop through the camera, and frame the video to obtain pictures; further, extract a picture from the video every 10 frames, and the extracted pictures include the standard wearing of the staff Pictures of hard hats and scenes without human bodies;
[0054] Step b. Use the camera to collect the video of the staff in the workshop without wearing a helmet, and frame the video to obtain a picture; that i...
Embodiment 2
[0065] This embodiment is further optimized on the basis of Embodiment 1. In the traditional YOLOv3 algorithm, the mean square error is generally used as the target positioning loss function to perform the regression of the detection frame, but the loss function based on the mean square error is very sensitive to scale information. Its partial derivative value will become very small when the output probability value is close to 0 or close to 1, which will easily cause the partial derivative value at the beginning of YOLOv3 model training to almost disappear, which will cause slow or even stagnant model training.
[0066] Therefore, in the present invention, the loss function is improved, and the logarithmic loss of the IoU value between the scale prediction frame and the real target frame is used to construct a loss function to measure the similarity between the scale prediction frame and the real target frame, effectively avoiding the loss function Sensitivity to scale informa...
Embodiment 3
[0077] This embodiment is further optimized on the basis of the above-mentioned embodiment 1 or 2. The feature network in the YOLOv3 model uses the multi-scale target detection network Darknet-53 for feature extraction, and the multi-scale target detection network Darknet-53 structure contains alternating occurrences of The 1×1 and 3×3 convolutional layers use the residual structure and the full convolutional network to solve the problem of gradient disappearance under the deep network, which reduces the difficulty of network training. In the original Darknet-53 network, the Softmax classifier is used to obtain the final output. In the improved multi-scale target detection network Darknet-53, the present invention expands the 3-scale detection of the original algorithm to 4-scale detection, performs 6 double downsampling and convolution in turn, and passes the 3rd, 3rd The 4th, 5th, and 6th times of double downsampling respectively obtain feature maps with sizes of 104×104 pix...
PUM
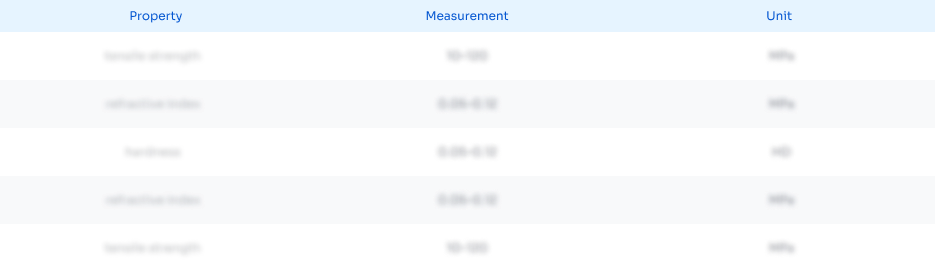
Abstract
Description
Claims
Application Information
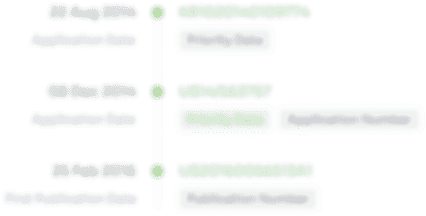
- R&D Engineer
- R&D Manager
- IP Professional
- Industry Leading Data Capabilities
- Powerful AI technology
- Patent DNA Extraction
Browse by: Latest US Patents, China's latest patents, Technical Efficacy Thesaurus, Application Domain, Technology Topic, Popular Technical Reports.
© 2024 PatSnap. All rights reserved.Legal|Privacy policy|Modern Slavery Act Transparency Statement|Sitemap|About US| Contact US: help@patsnap.com