Residual oil distribution prediction method and device based on deep convolutional neural network
A neural network and distribution prediction technology, which is applied in neural learning methods, biological neural network models, predictions, etc., can solve the problems of inability to predict the distribution of remaining oil saturation, low prediction efficiency of the method, and no reduction in the amount of calculation, etc., to achieve a strong general The ability to optimize, reduce time costs, and predict the effect of high accuracy
- Summary
- Abstract
- Description
- Claims
- Application Information
AI Technical Summary
Problems solved by technology
Method used
Image
Examples
Embodiment Construction
[0076] Specific embodiments of the present invention will be described in detail below in conjunction with the accompanying drawings. It should be understood that the specific embodiments described here are only used to illustrate and explain the present invention, and are not intended to limit the present invention.
[0077] figure 1 It is a flowchart of a method for predicting remaining oil distribution based on a deep convolutional neural network provided by an embodiment of the present invention. Such as figure 1 As shown, the forecasting method includes:
[0078] Establish a training data set according to the geological parameters and development parameters of the type of reservoir to which the target reservoir belongs;
[0079] Using the training data set to train and obtain a deep fully convolutional encoding and decoding neural network prediction model;
[0080] The remaining oil distribution of the target reservoir at any time is predicted by using the deep full c...
PUM
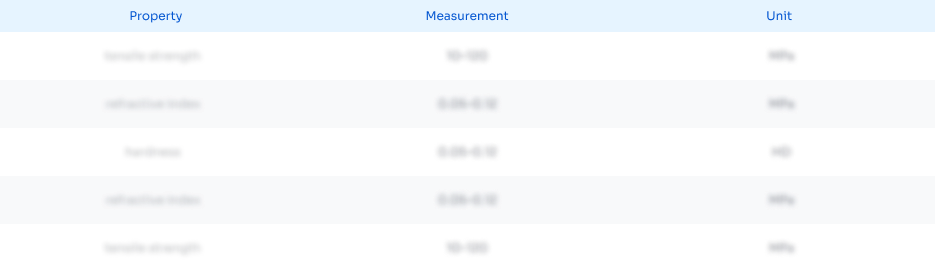
Abstract
Description
Claims
Application Information
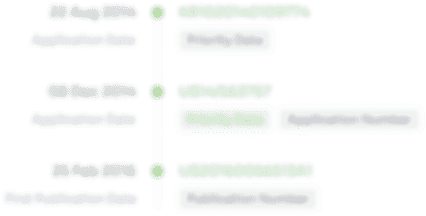
- R&D Engineer
- R&D Manager
- IP Professional
- Industry Leading Data Capabilities
- Powerful AI technology
- Patent DNA Extraction
Browse by: Latest US Patents, China's latest patents, Technical Efficacy Thesaurus, Application Domain, Technology Topic, Popular Technical Reports.
© 2024 PatSnap. All rights reserved.Legal|Privacy policy|Modern Slavery Act Transparency Statement|Sitemap|About US| Contact US: help@patsnap.com