Regional landslide susceptibility prediction method based on semi-supervised random forest model
A technology of random forest model and prediction method, applied in prediction, computational model, machine learning and other directions, can solve the problems of reducing the accuracy, difficulty and high cost of landslide susceptibility prediction, and improve the prediction modeling of landslide susceptibility. Performance, wide-ranging representative effects
- Summary
- Abstract
- Description
- Claims
- Application Information
AI Technical Summary
Problems solved by technology
Method used
Image
Examples
Embodiment Construction
[0034] The invention discloses a method for predicting regional landslide susceptibility based on a semi-supervised random forest model, comprising the following steps:
[0035]The object of the present invention is to realize by a kind of regional landslide susceptibility prediction method based on semi-supervised random forest model, comprises the following steps:
[0036] S1: Manage and spatially analyze landslide catalogs and related control factors in the study area through RS and ArcGIS platforms. The known landslide samples, the control factors are at least one of topography, basic geology, hydrological environment, and land cover data;
[0037] The quality of landslide catalog data has a very important impact on the performance of susceptibility prediction in a study area. The cataloging of landslides is helpful to understand the location, movement type, triggering times, scale and related geological environment development status of landslides and other information. ...
PUM
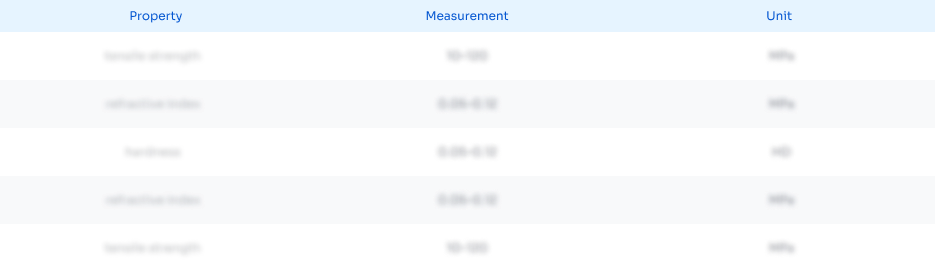
Abstract
Description
Claims
Application Information
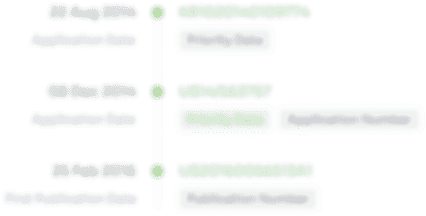
- R&D Engineer
- R&D Manager
- IP Professional
- Industry Leading Data Capabilities
- Powerful AI technology
- Patent DNA Extraction
Browse by: Latest US Patents, China's latest patents, Technical Efficacy Thesaurus, Application Domain, Technology Topic.
© 2024 PatSnap. All rights reserved.Legal|Privacy policy|Modern Slavery Act Transparency Statement|Sitemap