Vehicle trajectory prediction method based on global attention and state sharing
A trajectory prediction and vehicle trajectory technology, applied in the field of automatic driving, can solve the problems of ghost traffic jams, time-consuming, traffic congestion, etc., and achieve the effect of high-precision prediction
- Summary
- Abstract
- Description
- Claims
- Application Information
AI Technical Summary
Problems solved by technology
Method used
Image
Examples
Embodiment
[0026] Such as Figure 1 to Figure 3 As shown, a vehicle trajectory prediction method based on global attention and state sharing includes the following steps:
[0027] (S1) A GAS-LED trajectory prediction model using an encoder-decoder LSTM model with global attention mechanism and state sharing; that is, the global attention mechanism is applied to assign weights to encoder state vectors to reflect the importance of different time steps, While avoiding the complexity of the model. Considering the excellent performance of attention mechanism in performing sequence prediction, we use the basic structure of global attention mechanism to directly extract key feature information encoding of historical trajectories. In order to improve the convergence efficiency of the model
[0028] (S2) In the GAS-LED trajectory prediction model, an encoder and decoder state sharing mechanism is used to reduce the computational workload, and two parallel computing GAS-LED trajectory prediction...
PUM
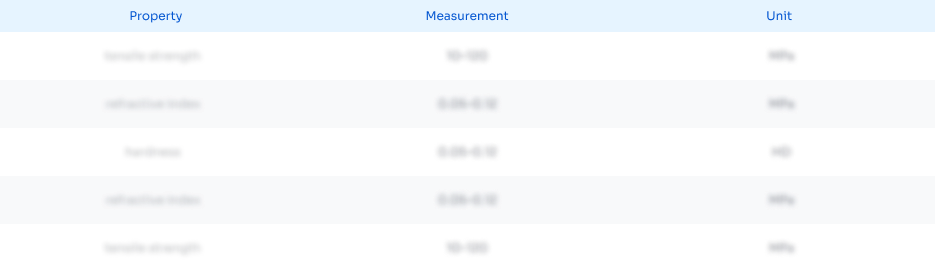
Abstract
Description
Claims
Application Information
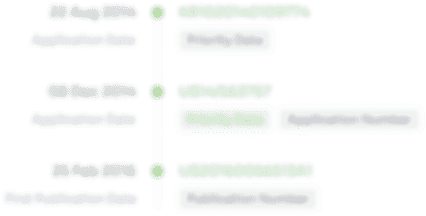
- R&D Engineer
- R&D Manager
- IP Professional
- Industry Leading Data Capabilities
- Powerful AI technology
- Patent DNA Extraction
Browse by: Latest US Patents, China's latest patents, Technical Efficacy Thesaurus, Application Domain, Technology Topic, Popular Technical Reports.
© 2024 PatSnap. All rights reserved.Legal|Privacy policy|Modern Slavery Act Transparency Statement|Sitemap|About US| Contact US: help@patsnap.com