Automobile longitudinal multi-state control method based on deep reinforcement learning priority extraction
A technology that reinforces learning and control methods, applied in design optimization/simulation, instrumentation, geometric CAD, etc., can solve problems such as inability to apply to various driving environments, sensor misjudgment, manual control, etc., to achieve good control stability and good control. Smooth, efficient effect
- Summary
- Abstract
- Description
- Claims
- Application Information
AI Technical Summary
Problems solved by technology
Method used
Image
Examples
Embodiment Construction
[0058]In this embodiment, a longitudinal polymorphic control method based on deep reinforced learning priority can decide the throttle opening degree and the main cylinder pressure of the car corresponding time according to the real-time state parameters of the car, thereby completing the car in high-speed state. Car driving, adaptive cruise, medium speed state emergency brake, low speed status - stopped polymorphism, specifically, as follows:
[0059]Step 1: Establish a vehicle dynamics model and vehicle driving environment model using Carsim software;
[0060]Step 2: Collect the car driving data in the real driving scene and act as an initialization data, the car driving data is the initial state information of the vehicle and the initial control parameter information of the vehicle;
[0061]Step 3: Define the status information set of the vehicle S = {S0, S1, ... st, ..., sn}, S0Indicates the initial status information of the vehicle, StIndicates that the vehicle is in the state St-1That ...
PUM
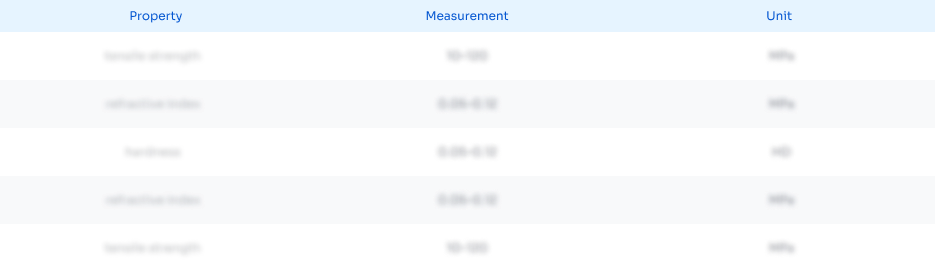
Abstract
Description
Claims
Application Information
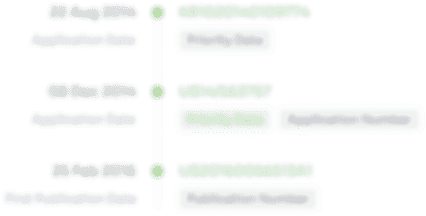
- R&D Engineer
- R&D Manager
- IP Professional
- Industry Leading Data Capabilities
- Powerful AI technology
- Patent DNA Extraction
Browse by: Latest US Patents, China's latest patents, Technical Efficacy Thesaurus, Application Domain, Technology Topic.
© 2024 PatSnap. All rights reserved.Legal|Privacy policy|Modern Slavery Act Transparency Statement|Sitemap