A Hybrid Flow Shop Scheduling Method Based on Temporal Difference
A workshop scheduling and pipeline technology, applied in data processing applications, instruments, biological neural network models, etc., can solve problems such as poor real-time performance, limited functions of function generalizers, difficulty in dealing with large-scale complex and changeable, and avoid excessive estimated effect
- Summary
- Abstract
- Description
- Claims
- Application Information
AI Technical Summary
Problems solved by technology
Method used
Image
Examples
Embodiment
[0083] Parameter selection may affect the solution quality, and there are general principles to follow. The discount factor γ measures the weight of the subsequent state value on the total return, so the general value is close to 1, set γ=0.95; in the ε-greedy strategy, ε should be changed from large to small first, so as to fully explore the strategy space in the initial stage, and the end stage Use the obtained optimal strategy, so the initial ε=1, and decay exponentially with a discount rate of 0.995; set the learning rate α=0.02, the maximum number of interactions MAX_EPISODE=1000; memory D capacity N=6000, sampling batch BATCH_SIZE=256; intelligent Volume convolutional neural network structure such as image 3 As shown, the network parameters adopt a random initialization strategy.
[0084] (1) Small-scale problems
[0085] Small-scale problems Take a 10×8×6 scheduling problem as an example to test the feasibility of the algorithm. The example contains 10 workpieces an...
PUM
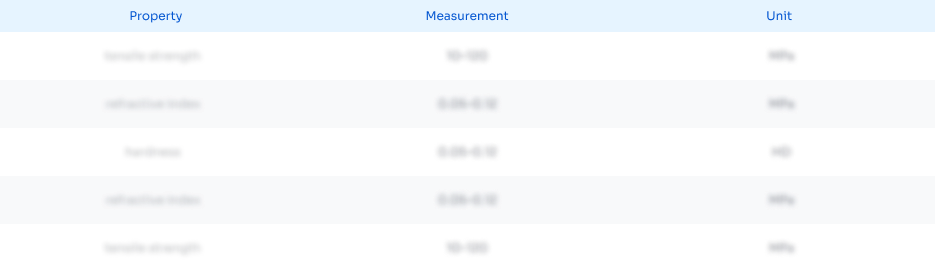
Abstract
Description
Claims
Application Information
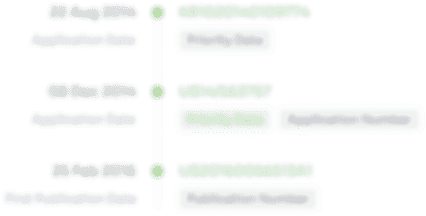
- R&D Engineer
- R&D Manager
- IP Professional
- Industry Leading Data Capabilities
- Powerful AI technology
- Patent DNA Extraction
Browse by: Latest US Patents, China's latest patents, Technical Efficacy Thesaurus, Application Domain, Technology Topic, Popular Technical Reports.
© 2024 PatSnap. All rights reserved.Legal|Privacy policy|Modern Slavery Act Transparency Statement|Sitemap|About US| Contact US: help@patsnap.com