Deep neural network-based multi-part reactive solute transport parameter inversion method
A deep neural network and multi-component response technology, applied in neural learning methods, biological neural network models, neural architectures, etc., can solve problems such as parameter identification
- Summary
- Abstract
- Description
- Claims
- Application Information
AI Technical Summary
Problems solved by technology
Method used
Image
Examples
Embodiment
[0063] This example is a hypothetical experimental model of a reactive solute migration simulation column in porous media, with a length of 0.08 m. The solute in the initial state solution is mainly 1mmol / L NaNO 3 and 0.2mmol / LKNO 3 . One end of the column is injected with 0.6mmol / L CaCl at a flow rate of 0.1m / h 2 solution. The porosity of the porous medium is 0.3, and the permeability is 7.0×10-12m 2 , the density is 2650kg / m 3 . The main chemical reaction in this system is the cation exchange process, and its expressions are mainly as follows:
[0064] Na + +K-X=K + +Na-X;
[0065] Na + +0.5Ca-X 2 =0.5Ca 2+ +Na-X;
[0066] K + and Ca 2+ Take Na + The cation exchange coefficients for reference are: K Na / K = 0.1995 and K Na / Ca =0.3981, the cation exchange capacity is 0.01779meq / 100g.
[0067] The initial concentration and boundary concentration of each ion are shown in Table 1:
[0068] Table 1 The initial concentration and boundary concentration of various...
PUM
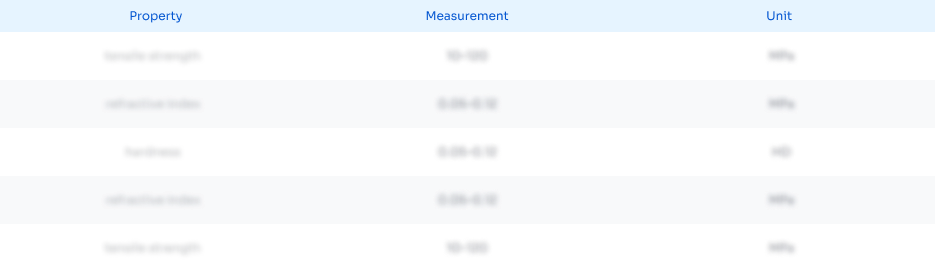
Abstract
Description
Claims
Application Information
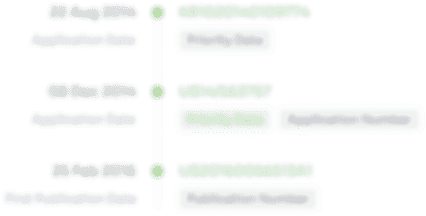
- Generate Ideas
- Intellectual Property
- Life Sciences
- Materials
- Tech Scout
- Unparalleled Data Quality
- Higher Quality Content
- 60% Fewer Hallucinations
Browse by: Latest US Patents, China's latest patents, Technical Efficacy Thesaurus, Application Domain, Technology Topic, Popular Technical Reports.
© 2025 PatSnap. All rights reserved.Legal|Privacy policy|Modern Slavery Act Transparency Statement|Sitemap|About US| Contact US: help@patsnap.com