Distributed machine learning task online scheduling method based on side cloud cooperation
A technology for machine learning and scheduling methods, applied in machine learning, instrumentation, program startup/switching, etc., can solve problems such as low latency, inability to capture edge network characteristics, and limited edge server resources.
- Summary
- Abstract
- Description
- Claims
- Application Information
AI Technical Summary
Problems solved by technology
Method used
Image
Examples
Embodiment Construction
[0052] The technical solutions in the embodiments of the present invention will be clearly and completely described below in conjunction with the accompanying drawings in the embodiments of the present invention. Obviously, the described embodiments are only some of the embodiments of the present invention, not all of them. Based on the embodiments of the present invention, all other embodiments obtained by persons of ordinary skill in the art without creative work all belong to the protection scope of the present invention.
[0053] see Figure 1-4 , an online scheduling method for distributed machine learning tasks based on edge-cloud collaboration. In this embodiment, we use S=200 edge servers, each edge server is equipped with 0:5 workers and 0:3 PSs, The types of worker and PS are set to 8:10, and their bandwidths are set to [100,5*1024]Mbps and [5,20]Gbps respectively. The configuration of the ML task is as follows: E j ∈[50,100],D j ∈[50,100], B j ∈[10,50],m j ∈[0....
PUM
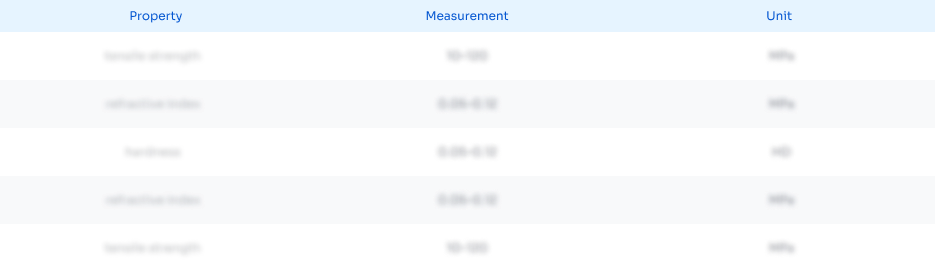
Abstract
Description
Claims
Application Information
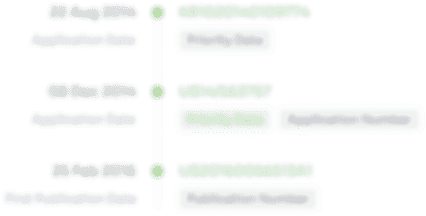
- R&D
- Intellectual Property
- Life Sciences
- Materials
- Tech Scout
- Unparalleled Data Quality
- Higher Quality Content
- 60% Fewer Hallucinations
Browse by: Latest US Patents, China's latest patents, Technical Efficacy Thesaurus, Application Domain, Technology Topic, Popular Technical Reports.
© 2025 PatSnap. All rights reserved.Legal|Privacy policy|Modern Slavery Act Transparency Statement|Sitemap|About US| Contact US: help@patsnap.com