Anomaly data detection method of server running network traffic based on small sample learning
A technology for network traffic and traffic anomalies, applied to biological neural network models, instruments, computing, etc., can solve problems such as server attacks, detection models to ensure network security, and data volume differences, and achieve the effect of reducing attacks
- Summary
- Abstract
- Description
- Claims
- Application Information
AI Technical Summary
Problems solved by technology
Method used
Image
Examples
Embodiment Construction
[0059] In order to illustrate the technical solution and content of the present invention more clearly, the present invention will be further described in detail below in conjunction with the accompanying drawings.
[0060] In the present invention, the network flow data recorded during the running of the server includes normal network flow data and two abnormal data of Satan type and Ipsweep type. Use the WireShark filter to filter multiple network traffic data in the traffic generator, denoted as normal-flow set FW, and FW={fw 1 , fw 2 ,..., fw a ,..., fw A}. Use the WireShark filter to filter multiple network traffic data in the attacking host, record it as anomaly-flow set HW, and HW={hw 1 ,hw 2 ,...,hw b ,...,hw B}.
[0061] fw 1 Indicates the first normal network traffic data; the fw 1 The network data packet carried, denoted as
[0062] fw 2 Indicates the second normal network traffic data; the fw 2 The network data packet carried, denoted as
[0063] ...
PUM
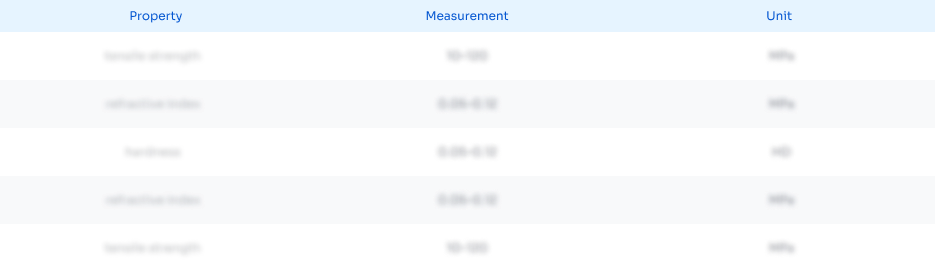
Abstract
Description
Claims
Application Information
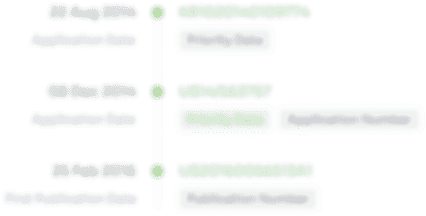
- R&D Engineer
- R&D Manager
- IP Professional
- Industry Leading Data Capabilities
- Powerful AI technology
- Patent DNA Extraction
Browse by: Latest US Patents, China's latest patents, Technical Efficacy Thesaurus, Application Domain, Technology Topic, Popular Technical Reports.
© 2024 PatSnap. All rights reserved.Legal|Privacy policy|Modern Slavery Act Transparency Statement|Sitemap|About US| Contact US: help@patsnap.com