Lightweight multi-modal sentiment analysis method based on multi-element hierarchical deep fusion
A sentiment analysis, multi-element technology, applied in neural learning methods, character and pattern recognition, biological neural network models, etc., can solve problems such as lack of multimodal characteristics
- Summary
- Abstract
- Description
- Claims
- Application Information
AI Technical Summary
Problems solved by technology
Method used
Image
Examples
Embodiment 1
[0043] like figure 1 As shown, the lightweight multi-modal sentiment analysis method based on multi-element layered deep fusion, the specific steps are as follows:
[0044] Step 1. Data matrix representation of a single modality
[0045] The data of multiple modalities involved in the present invention are represented in the form of X, Y and Z respectively. In the actual use of the framework, three types of modal data are used: text modal, visual modal and acoustic modal to perceive the emotional state of the subjects. We refer to the text mode with a capital letter Y, the visual mode with a capital letter X, and the acoustic mode with a capital letter Z. The data of each modality can be organized in the form of a two-dimensional matrix, namely Among them, t x , t y , t z Respectively represent the number of three modal elements, d x 、d y 、d z represent the characteristic lengths of the corresponding elements, respectively. Taking the text mode as an example, the su...
Embodiment 2
[0097] The difference between this embodiment and Embodiment 1 is that the lightweight multi-modal sentiment analysis method based on multi-element layered deep fusion is applied to four modal data (for example: text modal, visual modal, acoustic modal and EEG data), we can also calculate the matching matrix between the two modalities first, and then use our proposed Ex-KR addition to integrate the matching matrix representing the local fusion of the two modalities to obtain a multimodal global tensor express. In order to illustrate the calculation process, the four modalities participating in the fusion can be denoted by m 1 , m 2 , m 3 , m 4 To represent, the corresponding modal data is organized into a two-dimensional matrix, that is, and In the same way as in equations (1) and (2), the context within a single modality can be captured using the bidirectional long-short-term memory network layer transformation LSTM( ) and the nonlinear feed-forward fully-connected lay...
PUM
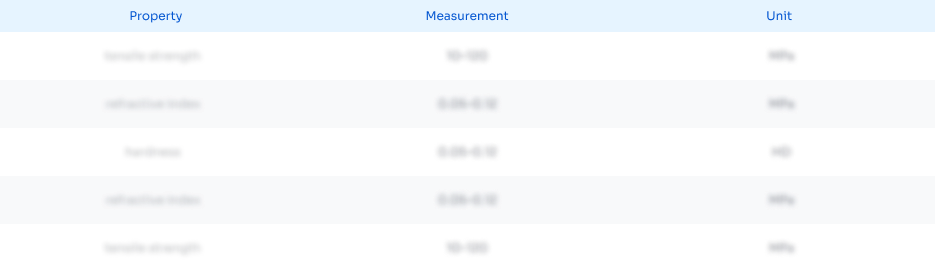
Abstract
Description
Claims
Application Information
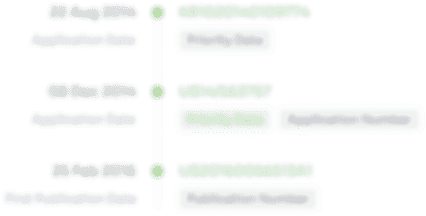
- R&D Engineer
- R&D Manager
- IP Professional
- Industry Leading Data Capabilities
- Powerful AI technology
- Patent DNA Extraction
Browse by: Latest US Patents, China's latest patents, Technical Efficacy Thesaurus, Application Domain, Technology Topic, Popular Technical Reports.
© 2024 PatSnap. All rights reserved.Legal|Privacy policy|Modern Slavery Act Transparency Statement|Sitemap|About US| Contact US: help@patsnap.com