Multi-base-station cooperative wireless network resource allocation method based on graph attention mechanism reinforcement learning
A technology of wireless network resources and reinforcement learning, applied in the field of multi-base station cooperative wireless network resource allocation based on graph attention mechanism reinforcement learning, can solve problems such as inability to cope well, lack of flexibility and scalability, etc.
- Summary
- Abstract
- Description
- Claims
- Application Information
AI Technical Summary
Problems solved by technology
Method used
Image
Examples
Embodiment Construction
[0041] In order to describe in detail the technical content, structural features, achieved goals and effects of the technical solution, the following will be described in detail in conjunction with the accompanying drawings.
[0042] refer to figure 1 , is a flow chart of the multi-base station cooperative wireless network resource allocation method based on graph attention mechanism reinforcement learning of the present invention, specifically including the following steps:
[0043] S1. Algorithm network structure G and target network Build and initialize, including the following sub-steps:
[0044] S11. The algorithm network structure G of this method includes three parts: a state vector encoding network (Embed), a graph attention mechanism network (GAT) and a deep Q network (DQN).
[0045] S12. The state vector encoding network is composed of two layers of fully connected networks, denoted as
[0046] , (1)
[0047] in , is the weight matrix of the layer, is th...
PUM
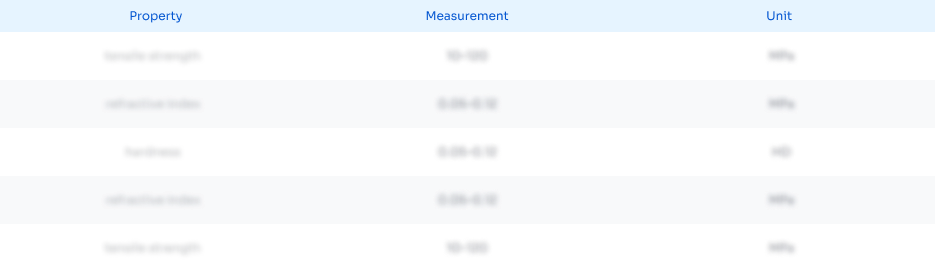
Abstract
Description
Claims
Application Information
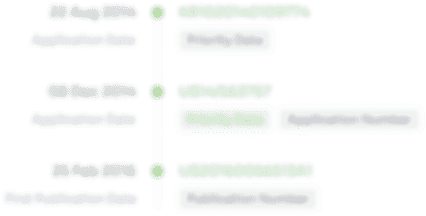
- R&D Engineer
- R&D Manager
- IP Professional
- Industry Leading Data Capabilities
- Powerful AI technology
- Patent DNA Extraction
Browse by: Latest US Patents, China's latest patents, Technical Efficacy Thesaurus, Application Domain, Technology Topic, Popular Technical Reports.
© 2024 PatSnap. All rights reserved.Legal|Privacy policy|Modern Slavery Act Transparency Statement|Sitemap|About US| Contact US: help@patsnap.com