Food inversion method based on multi-task learning and attention mechanism
A multi-task learning and attention technology, applied in the field of image recognition, can solve the problems of low calorie prediction accuracy, dependence on ingredients and cooking methods, etc.
- Summary
- Abstract
- Description
- Claims
- Application Information
AI Technical Summary
Problems solved by technology
Method used
Image
Examples
Embodiment
[0144] This system directly predicts the calorie value of a food image, without the need for the user to manually input other information, does not require the image shooting angle, etc., does not need to use special equipment such as depth cameras, and the user operation is more convenient.
[0145] Such as Figure 9 , Figure 10 As shown, the model adopts a multi-task convolutional neural network, and simultaneously learns four tasks of calorie prediction, food classification, ingredient prediction and recipe prediction during training, which effectively improves the accuracy of food classification and calorie prediction; the experimental results show that the present invention Compared with the single-task convolutional neural network calorie prediction model (correlation coefficient = 0.7217), the correlation coefficient of the proposed model (correlation coefficient = 0.7679) was improved by 0.0462.
[0146] The invention constructs a food data set, which contains 281 fi...
PUM
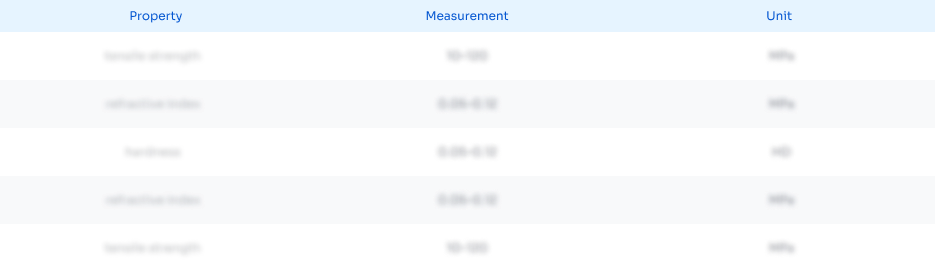
Abstract
Description
Claims
Application Information
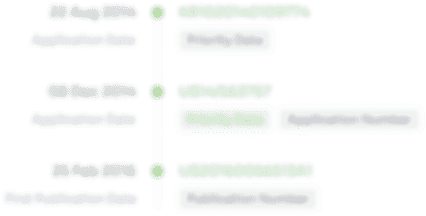
- R&D Engineer
- R&D Manager
- IP Professional
- Industry Leading Data Capabilities
- Powerful AI technology
- Patent DNA Extraction
Browse by: Latest US Patents, China's latest patents, Technical Efficacy Thesaurus, Application Domain, Technology Topic, Popular Technical Reports.
© 2024 PatSnap. All rights reserved.Legal|Privacy policy|Modern Slavery Act Transparency Statement|Sitemap|About US| Contact US: help@patsnap.com