Melody MIDI accompaniment generation method based on deep neural network
A deep neural network and melody technology, applied in neural learning methods, biological neural network models, neural architectures, etc., can solve the problems of large number of parameters, low music quality, high time cost, short generation time, high music quality, The effect of less hardware resources
- Summary
- Abstract
- Description
- Claims
- Application Information
AI Technical Summary
Problems solved by technology
Method used
Image
Examples
Embodiment Construction
[0025] The invention discloses a method for generating a melody MIDI accompaniment based on a deep neural network, which specifically includes the following steps:
[0026] (1) Use crawlers to collect MIDI data sets with genre labels on the Internet, and classify them according to genre labels; the genres include: popular, country, jazz; channels for collecting MIDI data sets include FreeMidi website, Lakh Midi Dataset Public dataset, MidiShow website.
[0027] (2) After the MIDI data collected in step (1) is extracted through melody, track compression, data filtering, whole song segmentation, and chord recognition, the MIDI fragments are obtained, and the MIDI fragments are disrupted to obtain a data set; the specific processing process like figure 1 As shown, including the following sub-steps:
[0028] (2.1) Melody extraction: using an open source tool: Midi Miner, the function of this tool is to analyze which track in a multi-track Midi is the melody track. Use Midi Mine...
PUM
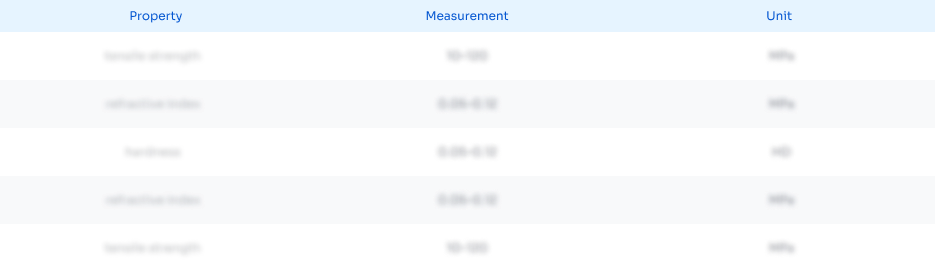
Abstract
Description
Claims
Application Information
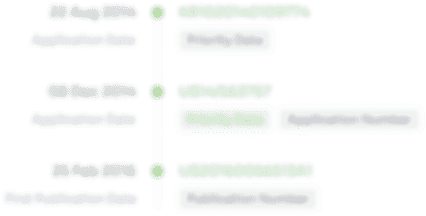
- R&D Engineer
- R&D Manager
- IP Professional
- Industry Leading Data Capabilities
- Powerful AI technology
- Patent DNA Extraction
Browse by: Latest US Patents, China's latest patents, Technical Efficacy Thesaurus, Application Domain, Technology Topic, Popular Technical Reports.
© 2024 PatSnap. All rights reserved.Legal|Privacy policy|Modern Slavery Act Transparency Statement|Sitemap|About US| Contact US: help@patsnap.com