Traffic index time sequence identification method based on autoregressive differential moving average-convolutional neural network
A technology of convolutional neural network and moving average algorithm, which is applied in the field of identifying traffic index time series, can solve the problems of traffic index time series data distortion, insufficient pattern recognition ability and deformation of traffic index time series data, and achieve accurate prediction and model The effect of identifying and alleviating urban traffic pressure
- Summary
- Abstract
- Description
- Claims
- Application Information
AI Technical Summary
Problems solved by technology
Method used
Image
Examples
specific Embodiment approach
[0111] The method for generating traffic index time series described in step 0000 comprises:
[0112] Step 0200: Calculate the average traffic index at each moment according to the original traffic index data set.
[0113] Step 0400: Select the first continuous time series and the second continuous time series.
[0114] Among them, the second threshold k 2 ∈(l 2 *X,l 1 *X), l 2 is a positive real number and l 2 ≥1, preferably, l 2 is 1.5.
[0115] Step 0600: According to the traffic index raw data set, the first continuous time series and the second continuous time series, divide the traffic index raw data set.
[0116] Step 0600 further comprises:
[0117] Step 0620, if the number of moments in the first continuous time series is n t , then divide the traffic index data of the i-th day into n 0 time periods, preferably, n 0 for n 2 and n t function, more preferably, Indicates rounding down.
[0118] Optional, if there are multiple first continuous time serie...
PUM
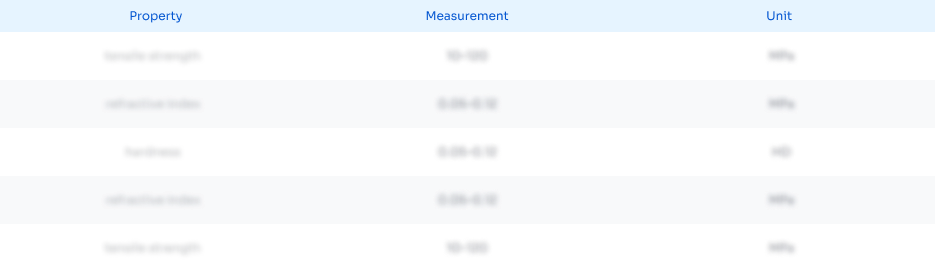
Abstract
Description
Claims
Application Information
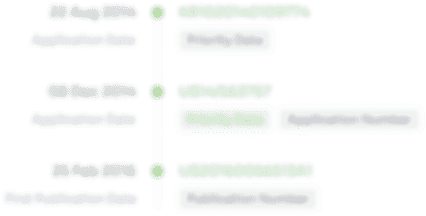
- R&D Engineer
- R&D Manager
- IP Professional
- Industry Leading Data Capabilities
- Powerful AI technology
- Patent DNA Extraction
Browse by: Latest US Patents, China's latest patents, Technical Efficacy Thesaurus, Application Domain, Technology Topic, Popular Technical Reports.
© 2024 PatSnap. All rights reserved.Legal|Privacy policy|Modern Slavery Act Transparency Statement|Sitemap|About US| Contact US: help@patsnap.com