Self-supervision low-illumination image enhancement and denoising method based on deep learning
A technology of image enhancement and deep learning, applied in image enhancement, image analysis, image data processing, etc., can solve problems such as inability to guarantee good contrast and difficulty in suppressing noise
- Summary
- Abstract
- Description
- Claims
- Application Information
AI Technical Summary
Problems solved by technology
Method used
Image
Examples
specific Embodiment approach 1
[0059] Specific implementation mode one: combine figure 1 To describe this embodiment,
[0060] A self-supervised low-light image enhancement and denoising method based on deep learning, comprising the following steps:
[0061] Step 1. Design an image enhancement network based on deep learning, which can decompose a low-light image into a reflection image R and an illumination image I;
[0062] The low-illuminance image S′ to be enhanced and its maximum channel image S′ max And its expected maximum channel image S' expect_max The matrix merged into M*N*5 is used as the input of the self-supervised low-light image enhancement network, and the low-light image is enhanced and denoised using the trained self-supervised low-light image enhancement network, and the output is the reflection image R and the illumination image I. The reflection image R output by the self-supervised low-light image enhancement network is the enhanced image.
[0063] The low-light image S' to be en...
specific Embodiment approach 2
[0100] A self-supervised low-light image enhancement and denoising method based on deep learning described in this embodiment, the specific process of the third step includes the following steps:
[0101] Step 31. Extract the maximum value channel image S of the low-illumination image S max
[0102]
[0103] Among them, S max (i, j) is the maximum channel image S max The i-th row and the j-th column element; max represents the maximum value operation; c is r, g, b, corresponding to the three color channels of red, green and blue in the rgb color space, S c (i,j) is the i-th row and j-th column element of a certain channel of the low-illuminance image S in the rgb color space;
[0104] Step 32, use any contrast enhancement method such as Gamma transform to the maximum value channel image S max Do a contrast enhancement operation to get the desired maximum channel image S expect_max .
[0105] Other steps and parameters are the same as those in the first embodiment.
specific Embodiment approach 3
[0106] Specific implementation mode three: combination figure 2 To describe this embodiment,
[0107] A self-supervised low-light image enhancement and denoising method based on deep learning described in this embodiment, the image enhancement network is specifically as follows:
[0108] The input is input to the first convolutional layer and the second convolutional layer respectively. The first convolutional layer and the second convolutional layer are respectively connected to a 9*9 convolutional layer and connected to an LReLU layer and a 3*3 convolutional layer. A LReLU layer;
[0109] The first convolutional layer is connected to the third convolutional unit, and the third convolutional unit is connected to an LReLU layer after the convolutional layer of 3*3;
[0110] The third convolution unit is connected to the fourth convolution unit, the fourth convolution unit is connected to the fifth convolution unit, the fifth convolution unit is connected to the sixth convol...
PUM
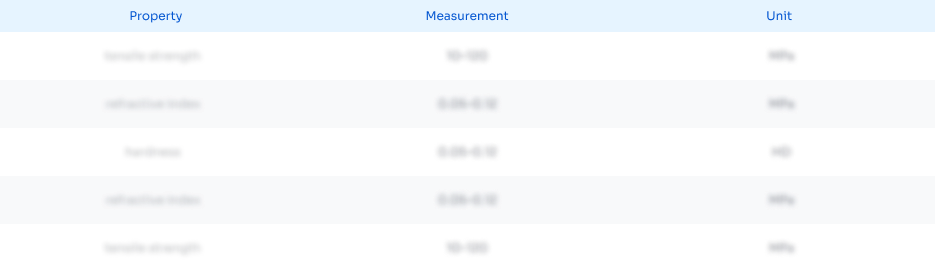
Abstract
Description
Claims
Application Information
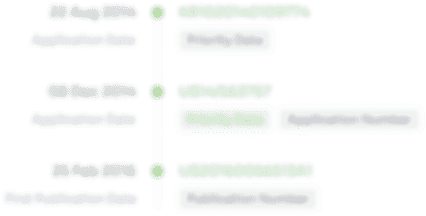
- R&D Engineer
- R&D Manager
- IP Professional
- Industry Leading Data Capabilities
- Powerful AI technology
- Patent DNA Extraction
Browse by: Latest US Patents, China's latest patents, Technical Efficacy Thesaurus, Application Domain, Technology Topic, Popular Technical Reports.
© 2024 PatSnap. All rights reserved.Legal|Privacy policy|Modern Slavery Act Transparency Statement|Sitemap|About US| Contact US: help@patsnap.com