Depth map convolution model defense method based on generative adversarial network
A convolution model and depth map technology, applied in the field of network security, can solve problems such as difficult to guarantee the effectiveness of defense, and achieve the effects of enhancing robustness, defending against attacks, and improving accuracy
- Summary
- Abstract
- Description
- Claims
- Application Information
AI Technical Summary
Problems solved by technology
Method used
Image
Examples
Embodiment Construction
[0019] In order to make the object, technical solution and advantages of the present invention clearer, the present invention will be further described in detail below in conjunction with the accompanying drawings and embodiments. It should be understood that the specific embodiments described here are only used to explain the present invention, and do not limit the protection scope of the present invention.
[0020] In order to enhance the robustness of deep graph convolutional models for classification tasks or recognition tasks of social networks, communication networks, biological networks or transaction networks to adversarial attacks, to improve the accuracy of classification tasks or recognition tasks. The embodiment provides a method for defending a deep graph convolution model based on a generative confrontation network, such as figure 1 As shown, the depth map convolution model defense method includes the following steps:
[0021] Step 1, build a multi-strategy gene...
PUM
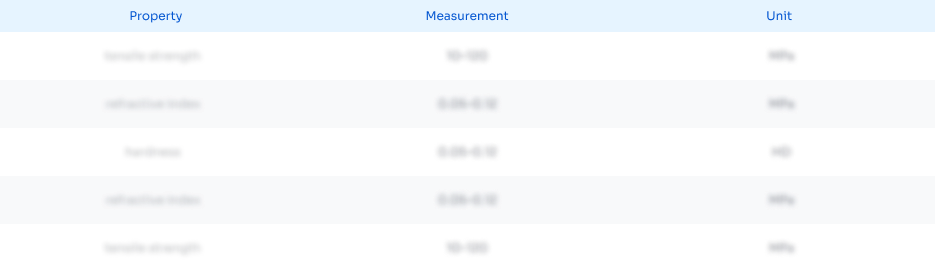
Abstract
Description
Claims
Application Information
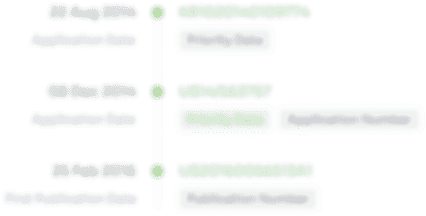
- R&D Engineer
- R&D Manager
- IP Professional
- Industry Leading Data Capabilities
- Powerful AI technology
- Patent DNA Extraction
Browse by: Latest US Patents, China's latest patents, Technical Efficacy Thesaurus, Application Domain, Technology Topic.
© 2024 PatSnap. All rights reserved.Legal|Privacy policy|Modern Slavery Act Transparency Statement|Sitemap