Consumption intention identification and prediction method based on consumption cause map
A prediction method and intent technology, applied in data processing applications, special data processing applications, other database retrieval, etc., can solve the problems of low accuracy of consumer intent recognition and prediction
- Summary
- Abstract
- Description
- Claims
- Application Information
AI Technical Summary
Problems solved by technology
Method used
Image
Examples
specific Embodiment approach 1
[0021] Specific implementation mode one: combine figure 1 This embodiment will be described. A method for identifying and predicting consumption intentions based on the consumer affairs map described in this embodiment is specifically implemented through the following steps:
[0022] Step 1. After fine-tuning the pre-trained BERT-Base model using the data marked with events, use the fine-tuned BERT-Base model to extract events from the narrative text;
[0023] Step 2. After fine-tuning the pre-trained BERT-Base model using the data marked with the event and the sequence relationship between events, use the fine-tuned BERT-Base model to make the sequence of the event pair composed of the events extracted in step 1. Identify the inheritance relationship and build a map of affairs;
[0024] Step 3. Construct a bipartite graph based on the events extracted in step 1 as weak supervision information;
[0025] Step 4. Using the bipartite graph embedding algorithm, combining the ev...
specific Embodiment approach 2
[0029] Embodiment 2: The difference between this embodiment and Embodiment 1 is that in the first step, the pre-trained BERT-Base model is fine-tuned using the data marked with events, and the specific process is as follows:
[0030] After inputting the labeled data into the pre-trained BERT-Base model, the fine-tuning process is carried out in two stages:
[0031] The first stage: set the learning rate to 1e-3, the training round is 2, the optimization algorithm used is the BertAdam algorithm, and the linear layer parameters of the pre-trained BERT-Base model are trained;
[0032] The second stage: set the learning rate to 3e-5, the training rounds to 10, the optimization algorithm used is the BertAdam algorithm, and the parameters of the linear layer and BERT layer of the pre-trained BERT-Base model are trained;
[0033] After completing the two-stage training, the fine-tuned BERT-Base model is obtained.
[0034] The annotation data used in this embodiment is the data after t...
specific Embodiment approach 3
[0036] Specific embodiment three: the difference between this embodiment and specific embodiment two is that in the second step, the pre-trained BERT-Base model is fine-tuned using the data marked with events and the sequential relationship between events. The process is:
[0037] After inputting the labeled data into the pre-trained BERT-Base model, the fine-tuning process is divided into three stages:
[0038] The first stage: train the parameters of the linear layer, the learning rate is set to 1e-3, the training round is 1, and the optimization algorithm used is the BertAdam algorithm;
[0039] The second stage: train the parameters of the linear layer and the event embedding layer, the learning rate is set to 5e-5, the training round is 3, and the optimization algorithm used is the BertAdam algorithm;
[0040] The third stage: train the parameters of all layers, the learning rate is set to 1e-5, the training round is 5, and the optimization algorithm used is the BertAdam...
PUM
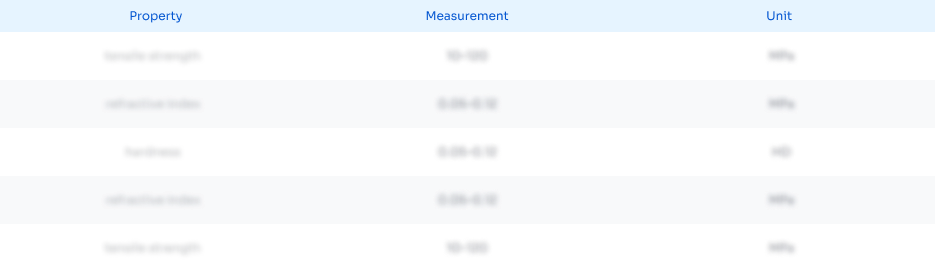
Abstract
Description
Claims
Application Information
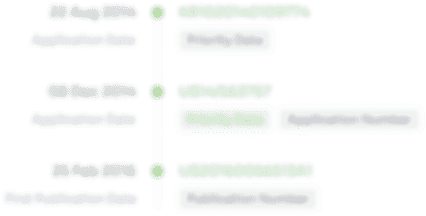
- R&D Engineer
- R&D Manager
- IP Professional
- Industry Leading Data Capabilities
- Powerful AI technology
- Patent DNA Extraction
Browse by: Latest US Patents, China's latest patents, Technical Efficacy Thesaurus, Application Domain, Technology Topic, Popular Technical Reports.
© 2024 PatSnap. All rights reserved.Legal|Privacy policy|Modern Slavery Act Transparency Statement|Sitemap|About US| Contact US: help@patsnap.com