Recommendation algorithm based on adversarial learning and bidirectional long-short-term memory network
A long-short-term memory and recommendation algorithm technology, applied in neural learning methods, biological neural network models, calculations, etc., can solve problems such as the influence of node representation
- Summary
- Abstract
- Description
- Claims
- Application Information
AI Technical Summary
Problems solved by technology
Method used
Image
Examples
Embodiment 1
[0088] see figure 1 , figure 2 , this embodiment discloses a recommendation algorithm based on adversarial learning and two-way long-short-term memory network,
[0089] Specifically include the following steps:
[0090] The first step, pre-defined symbols
[0091] A1) Definition of heterogeneous information network: use the symbol G=(V, E) to represent a heterogeneous information network, where V is a set of nodes, and E is a set of edges;
[0092] A2) Path definition in heterogeneous information network: there is a mapping relationship between each node v and each edge e in the heterogeneous network where T V and T E are node type set and edge type set respectively, T V ≥2 or T E ≥2, U represents the user set, u∈U represents the uth user, there are m users in total, I represents the item set, i∈I represents the i-th item, and there are n items in total;
[0093] A3) In the heterogeneous information network G, define the node connection sequence from user u to item i...
PUM
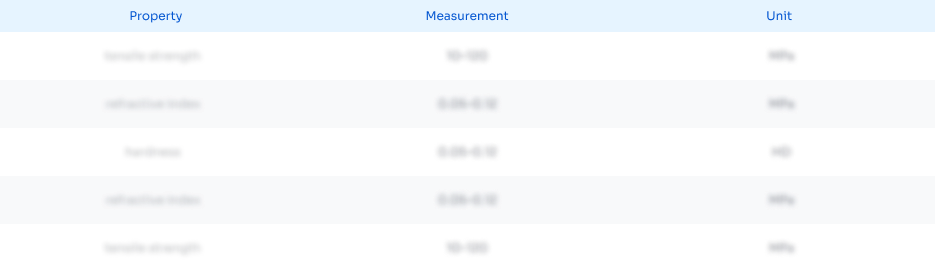
Abstract
Description
Claims
Application Information
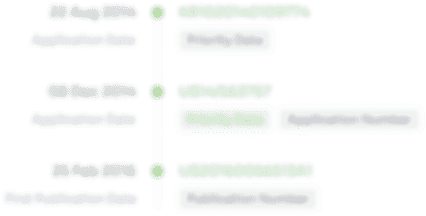
- R&D Engineer
- R&D Manager
- IP Professional
- Industry Leading Data Capabilities
- Powerful AI technology
- Patent DNA Extraction
Browse by: Latest US Patents, China's latest patents, Technical Efficacy Thesaurus, Application Domain, Technology Topic, Popular Technical Reports.
© 2024 PatSnap. All rights reserved.Legal|Privacy policy|Modern Slavery Act Transparency Statement|Sitemap|About US| Contact US: help@patsnap.com