Semi-supervised semantic segmentation model training method, semi-supervised semantic segmentation model identification method and semi-supervised semantic segmentation model identification device
A semantic segmentation and model training technology, applied in the field of image processing, can solve the problems of insufficient model generalization ability and affecting the accuracy of image recognition.
- Summary
- Abstract
- Description
- Claims
- Application Information
AI Technical Summary
Problems solved by technology
Method used
Image
Examples
Embodiment Construction
[0077] As mentioned above, in order to identify the object to be labeled in the image, it is necessary to train a machine model that can automatically identify the object to be labeled in the image. The data used to train the machine model is currently obtained through manual labeling. For example, if it is necessary to train and identify the types of crops and the geographical range of planting in remote sensing images, the existing technology is to manually label the objects to be marked in the image, that is, the geographical range of crop planting and the types of crops, and then use The manually labeled data is used as the data for training the machine model.
[0078] However, the ability to manually label images is limited, which will result in a small amount of data for training the model, and cannot generate a large amount of data for training the model, thus resulting in low accuracy of the model. For example, the trained model is usually only suitable for images tha...
PUM
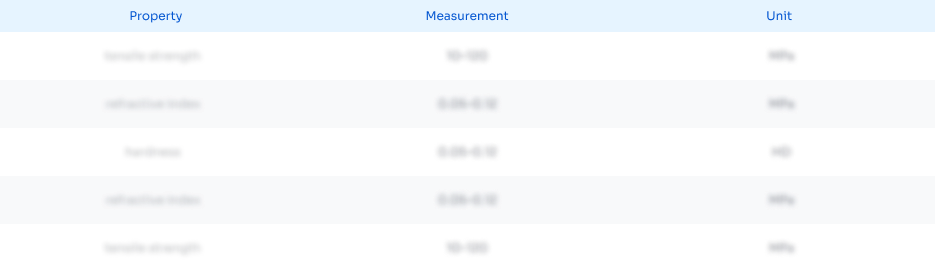
Abstract
Description
Claims
Application Information
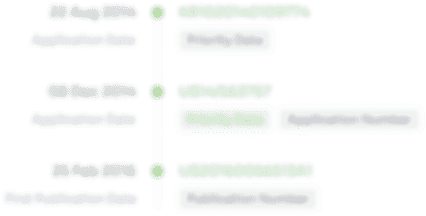
- R&D Engineer
- R&D Manager
- IP Professional
- Industry Leading Data Capabilities
- Powerful AI technology
- Patent DNA Extraction
Browse by: Latest US Patents, China's latest patents, Technical Efficacy Thesaurus, Application Domain, Technology Topic, Popular Technical Reports.
© 2024 PatSnap. All rights reserved.Legal|Privacy policy|Modern Slavery Act Transparency Statement|Sitemap|About US| Contact US: help@patsnap.com