Security scene flame detection method based on deep learning
A technology of deep learning and flame detection, which is applied in the field of flame detection in security scenes based on deep learning, can solve problems such as inability to obtain flame dynamic information, false alarms, and recognition of objects similar to flames as flames, etc.
- Summary
- Abstract
- Description
- Claims
- Application Information
AI Technical Summary
Problems solved by technology
Method used
Image
Examples
Embodiment 1
[0039] Such as Figure 1 ~ Figure 4As shown, the present invention is based on deep learning security scene flame detection method, comprising the following steps:
[0040] S1. A single-stage detection model for recognizing flame shapes is trained through a deep learning neural network;
[0041] S2. Train a class behavior recognition and classification model for recognizing flame dynamic changes through deep learning neural network;
[0042] S3. Return the video captured by the monitoring camera in real time to the background server;
[0043] S4. The background server decodes the returned video stream data into multiple frames of pictures;
[0044] S5. Input the picture obtained in step S4 into the single-stage detection model, whether the single-stage detection model detects whether there is a region suspected of flame, if not, repeat steps S3 and S4; if there is, then output the region suspected of flame in the figure;
[0045] S6. According to the suspected flame area id...
Embodiment 2
[0054] This embodiment is a specific description about the training of the single-stage detection model in Embodiment 1.
[0055] Said S1 specifically includes the following steps:
[0056] a. Data preparation: shooting and / or collecting flame videos;
[0057] b. Labeling: first use Opencv to decode the video into a picture, then use labeling software such as Labelimg or Labelme to mark out the flame in the picture, and use a rectangular frame to frame it, such as image 3 shown; and obtain the position (x, y, w, h) format of the flame in the image according to the annotation, where x, y are the coordinates of the upper left corner of the rectangular frame where the flame is located, and w and h are the width and height of the flame rectangular frame;
[0058] c. Training: Use the pure yolov3 full network or efficient-bo framework as the backbone, followed by the yolov3 lightweight detection head as the network structure of the single-stage detection model, and then use the m...
Embodiment 3
[0061] This embodiment is a specific description about the training of the class behavior recognition and classification model in the first embodiment.
[0062] Said S2 specifically includes the following steps:
[0063] A, data preparation: shooting and / or collecting flame video;
[0064] B. Annotation: Annotators mark the start frame and end frame of each video flame and the position of the flame;
[0065] C. Training: Use the ECO behavior recognition network structure as the network structure of the class behavior recognition classification model. Among the marked videos obtained in step B, the events from a flame occurrence to the end are counted as positive sample events, and those not marked as flames are long enough The video segment is recorded as a negative sample event, and 16 frames are sampled from both positive and negative sample events as network input, and the positive and negative events are used as labels to calculate the difference between the predicted res...
PUM
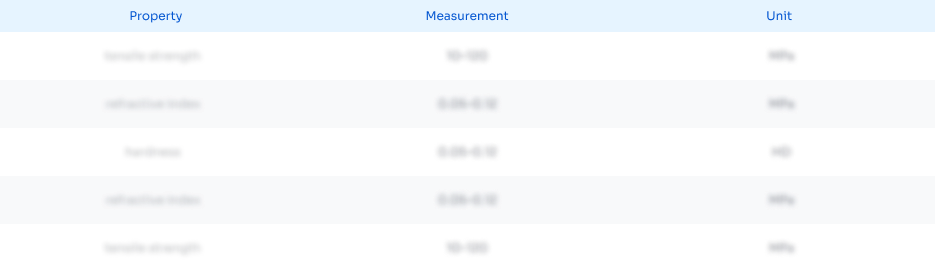
Abstract
Description
Claims
Application Information
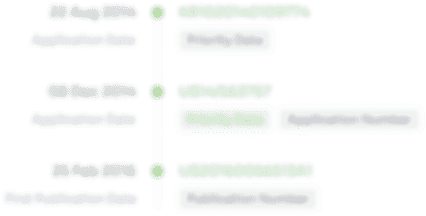
- Generate Ideas
- Intellectual Property
- Life Sciences
- Materials
- Tech Scout
- Unparalleled Data Quality
- Higher Quality Content
- 60% Fewer Hallucinations
Browse by: Latest US Patents, China's latest patents, Technical Efficacy Thesaurus, Application Domain, Technology Topic, Popular Technical Reports.
© 2025 PatSnap. All rights reserved.Legal|Privacy policy|Modern Slavery Act Transparency Statement|Sitemap|About US| Contact US: help@patsnap.com