Urban road traffic jam multi-reason automatic real-time identification method and system
A technology for road traffic and traffic congestion, applied in the field of traffic management, can solve the problems of time-consuming subjective judgment, low precision, and strong subjectivity.
- Summary
- Abstract
- Description
- Claims
- Application Information
AI Technical Summary
Problems solved by technology
Method used
Image
Examples
Embodiment 1
[0142] 1) for the 5 cause types studied in the present invention, they are respectively pedestrian impact, cross-street traffic impact, traffic peak, unreasonable signal timing and parking occupation of these 5 cause types, with reference to Quanxiu Street, Quanzhou City, Fujian Province Road to Tian'an Road direction), some roads are divided into three sections A, B, and C, and the road structure is as follows image 3 shown. The congestion cause type variable table is shown in Table 1, which is recorded as {c 1 ,c 2 ,c 3 ,c 4 ,c 5}. According to the road monitoring video, the observable traffic state variables on the road section are shown in Table 2.
[0143] 2) In order to meet the modeling requirements, continuous variables need to be treated as discrete variables. The settings of each variable are shown in Table 2. The data before setting are shown in Table 3, and the corresponding data after setting are shown in Table 4.
[0144] Table 2 Observable traffic state ...
Embodiment 2
[0155] Under the influence of various factors related to traffic congestion, the results of the parameter learning part of this model are shown in Table 5.
[0156] Table 5 Example of some results of parameter learning
[0157]
[0158]
Embodiment 3
[0160] The present invention uses a BP neural network model as a comparative experiment, wherein the number of hidden layer nodes is set to 13, the maximum number of training times is set to 300, and the maximum number of verification failure times is set to 50.
[0161] The average accuracy rate of the analysis results of the model constructed by the present invention and the comparison model on the impact of pedestrians, peak traffic flow, parking occupation, unreasonable signal timing and cross-street traffic flow is shown in Table 6.
[0162] Table 6 model accuracy
[0163] congestion type Bayesian network BP neural network Pedestrian impact 80.26% 56.55% rush hour 85.53% 71.88% parking in lane 81.58% 86.16% Signal timing is unreasonable 95.39% 89.49% The impact of cross-street traffic 87.50% 64.55%
[0164] According to the specific embodiments provided by the invention, the invention discloses the following technical e...
PUM
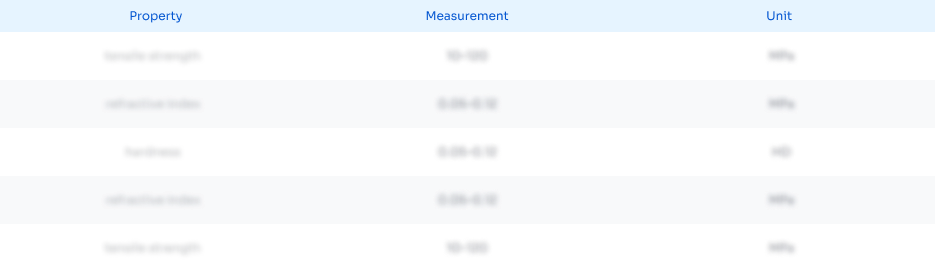
Abstract
Description
Claims
Application Information
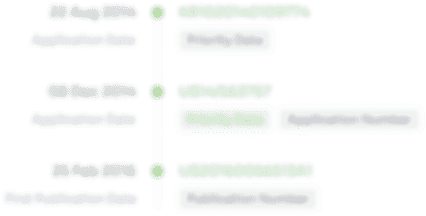
- R&D Engineer
- R&D Manager
- IP Professional
- Industry Leading Data Capabilities
- Powerful AI technology
- Patent DNA Extraction
Browse by: Latest US Patents, China's latest patents, Technical Efficacy Thesaurus, Application Domain, Technology Topic, Popular Technical Reports.
© 2024 PatSnap. All rights reserved.Legal|Privacy policy|Modern Slavery Act Transparency Statement|Sitemap|About US| Contact US: help@patsnap.com