Short-term power load prediction method based on CNN-IPSO-GRU hybrid model
A short-term load forecasting and hybrid model technology, applied in forecasting, computational models, biological models, etc., can solve the problems of large error results, reduced model prediction efficiency and accuracy, and achieve the goal of reducing losses and ensuring economical and reliable operation of the power grid. Effect
- Summary
- Abstract
- Description
- Claims
- Application Information
AI Technical Summary
Problems solved by technology
Method used
Image
Examples
Embodiment
[0082]In view of the fact that the factors affecting the load change of the power grid are complex and time-sequential, and the existing machine learning prediction method has the deficiency of selecting key parameters based on experience, this embodiment proposes an optimization method based on convolutional neural network and improved particle swarm optimization algorithm. The short-term load forecasting method of the gated recurrent unit firstly uses the convolutional neural network to extract the multidimensional feature vector representing the load change, and constructs a time series input to the gated recurrent unit network model; then uses the improved particle swarm optimization algorithm to analyze the gated recurrent unit The hyperparameters in the model (the number of neurons in the hidden layer and the learning rate) are optimized iteratively, and the optimal parameters are obtained under the premise of the highest prediction accuracy, and finally the short-term loa...
PUM
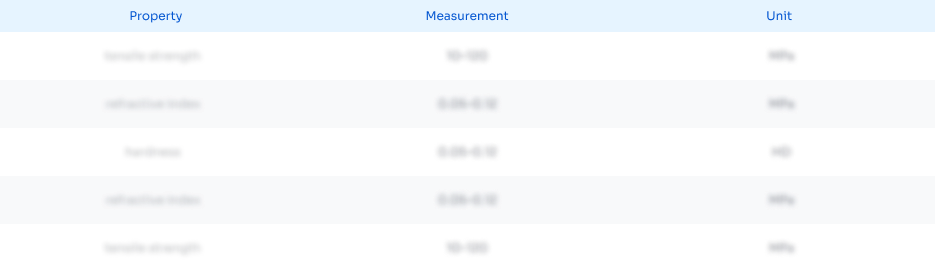
Abstract
Description
Claims
Application Information
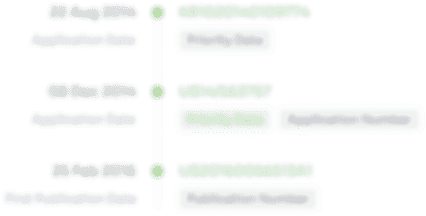
- R&D Engineer
- R&D Manager
- IP Professional
- Industry Leading Data Capabilities
- Powerful AI technology
- Patent DNA Extraction
Browse by: Latest US Patents, China's latest patents, Technical Efficacy Thesaurus, Application Domain, Technology Topic, Popular Technical Reports.
© 2024 PatSnap. All rights reserved.Legal|Privacy policy|Modern Slavery Act Transparency Statement|Sitemap|About US| Contact US: help@patsnap.com