Wind turbine bearing fault diagnosis method based on rapid multi-separation dictionary learning
A dictionary learning and fault diagnosis technology, applied in computer parts, character and pattern recognition, testing of mechanical parts, etc., can solve the problems of K_SVD, large atomic weight of dictionary, slow operation speed, etc.
- Summary
- Abstract
- Description
- Claims
- Application Information
AI Technical Summary
Problems solved by technology
Method used
Image
Examples
Embodiment
[0060] In this embodiment, the fault data of the rolling bearing of the direct-drive permanent magnet wind turbine are used for analysis. The structure of the wind turbine is figure 1 As shown, 1H is in the horizontal direction of the main bearing, 1V is in the vertical direction of the main bearing, and 1A is in the axial direction of the main bearing. The mechanical characteristic frequency of the wind turbine at rated power is shown in Table 1.
[0061] Table 1 Mechanical characteristic frequencies of wind turbine rear bearings
[0062]
[0063] The horizontal vibration (1H) signal of the rear bearing of the wind turbine is selected as the processing object. The time domain and frequency domain of the measured signal are as follows: Image 6 , as shown in 7. It is difficult to see the fault information from the picture. This embodiment provides a wind turbine bearing fault diagnosis method based on fast multi-separation dictionary learning, the flow chart is as follo...
PUM
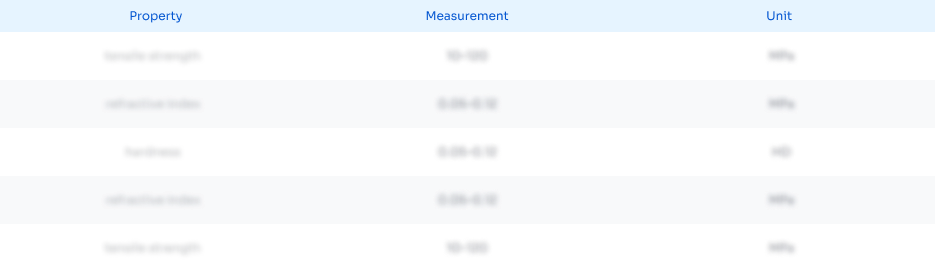
Abstract
Description
Claims
Application Information
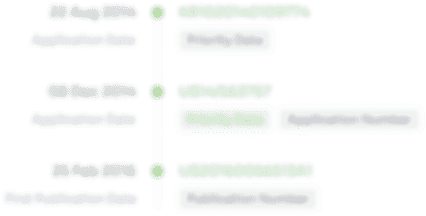
- R&D
- Intellectual Property
- Life Sciences
- Materials
- Tech Scout
- Unparalleled Data Quality
- Higher Quality Content
- 60% Fewer Hallucinations
Browse by: Latest US Patents, China's latest patents, Technical Efficacy Thesaurus, Application Domain, Technology Topic, Popular Technical Reports.
© 2025 PatSnap. All rights reserved.Legal|Privacy policy|Modern Slavery Act Transparency Statement|Sitemap|About US| Contact US: help@patsnap.com