GAN architecture and method for performing data augmentation on medical image data set based on generative adversarial network
A technology of medical imaging and network data, applied in the field of image processing, to avoid negative effects and improve accuracy
- Summary
- Abstract
- Description
- Claims
- Application Information
AI Technical Summary
Problems solved by technology
Method used
Image
Examples
Embodiment
[0032] Insufficient data sample size is a common problem in the process of obtaining medical imaging data sets, which is manifested in: the number of samples containing lesion information in the data set is far from the number of healthy samples; the number of samples of a certain modality is different from that of other samples The sample size of the modality is unbalanced, and the sample size of the data with obvious lesion information is too small. If the data set or algorithm is not improved accordingly, and the classification training is carried out directly, the result is that the sample data of the minority class will not be given sufficient attention, and in severe cases, it will even be ignored by the classifier as noise, resulting in poor classification results. Serious deviation.
[0033] In this context, how to augment the data samples with a small sample size in the medical imaging dataset and obtain our ideal results in the classifier has become a problem that re...
PUM
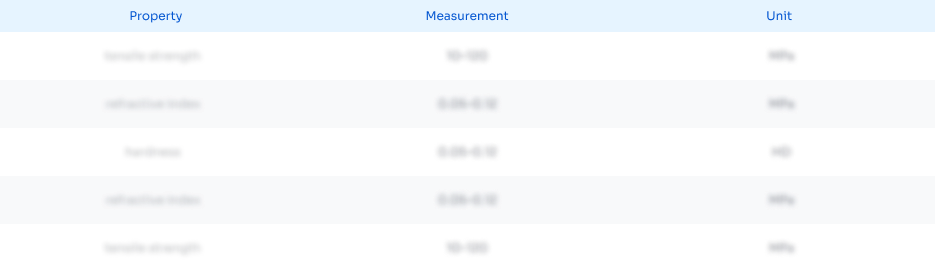
Abstract
Description
Claims
Application Information
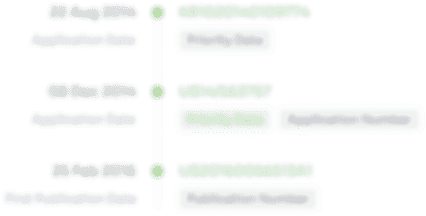
- R&D Engineer
- R&D Manager
- IP Professional
- Industry Leading Data Capabilities
- Powerful AI technology
- Patent DNA Extraction
Browse by: Latest US Patents, China's latest patents, Technical Efficacy Thesaurus, Application Domain, Technology Topic, Popular Technical Reports.
© 2024 PatSnap. All rights reserved.Legal|Privacy policy|Modern Slavery Act Transparency Statement|Sitemap|About US| Contact US: help@patsnap.com