Evaluation object-oriented sentiment analysis method for multi-task joint learning
A technology for evaluating objects and sentiment analysis, applied in text database clustering/classification, special data processing applications, instruments, etc., can solve the problems of low accuracy of text useful information extraction and sentiment evaluation word extraction without considering relevance, etc. The effect of joint extraction task optimal and good extraction effect
- Summary
- Abstract
- Description
- Claims
- Application Information
AI Technical Summary
Problems solved by technology
Method used
Image
Examples
specific Embodiment approach 1
[0023] Specific implementation manner 1: The specific process of an evaluation object-oriented sentiment analysis method for multi-task joint learning in this implementation manner is:
[0024] Step 1: The text is represented by word vectors;
[0025] Step 2: Represent the word vector of the text based on step 1. Perform a preliminary abstraction and get a shared representation;
[0026] Step 3: Perform evaluation object and evaluation word extraction based on step 2, and obtain the label probability distribution of evaluation object and evaluation word extraction;
[0027] Step 4: Perform emotional judgment of the evaluation object based on Step 2 and Step 3, and obtain the label probability distribution of the evaluation object's emotional judgment;
[0028] Step 5: Perform information transmission based on steps 3 and 4, and get the updated probability distribution prob AE , Prob AS ;
[0029] Step 6: Based on the model structure determined in steps 1 to 5, use artificial annotation...
specific Embodiment approach 2
[0030] Specific embodiment two: this embodiment is different from specific embodiment one in that: in the step one, the text is represented by word vectors; the specific process is:
[0031] The text X = {w 0 ,w 1 ,...,w n } Use domain word vector E domain And general word vector E general Is represented and spliced as
[0032] Among them, the domain word vector is trained on a large-scale unlabeled corpus in the target domain (for example, when the target domain is laptop, the data size used is 142.8M Amazon reviews on laptop);
[0033] The general word vector is trained on a large-scale unlabeled corpus in a large-scale indefinite field (text obtained from various websites containing 840B words (not limited to whether it is a comment));
[0034] E domain =Embedding domain (X) (1)
[0035] E general =Embedding general (X) (2)
[0036]
[0037] In the formula, X is the index of the input text, Embedding domain Is the domain word vector layer; E domain Represents the domain wor...
specific Embodiment approach 3
[0041] Specific embodiment three: this embodiment is different from specific embodiment one or two in that in the second step, the word vector of the text is expressed based on the step one Perform a preliminary abstraction to obtain a shared representation; the specific process is:
[0042] Use a single-layer CNN to represent the word vector of the text Perform a preliminary abstraction to get the shared representation output shared ;
[0043] The purpose is to block the sentence, so that the semantic structure such as the phrase is represented as a whole, and reduce the task amount of the subsequent task model;
[0044]
[0045] In the formula, output shared To abstract the shared representation, CNN shared It is a CNN layer used for preliminary abstraction to obtain a shared representation.
[0046] Other steps and parameters are the same as those in the first or second embodiment.
PUM
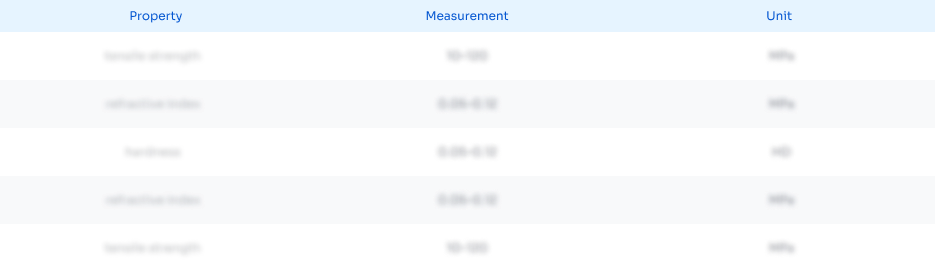
Abstract
Description
Claims
Application Information
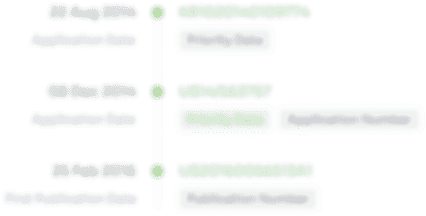
- R&D Engineer
- R&D Manager
- IP Professional
- Industry Leading Data Capabilities
- Powerful AI technology
- Patent DNA Extraction
Browse by: Latest US Patents, China's latest patents, Technical Efficacy Thesaurus, Application Domain, Technology Topic.
© 2024 PatSnap. All rights reserved.Legal|Privacy policy|Modern Slavery Act Transparency Statement|Sitemap