Natural language relation extraction method based on multi-task learning mechanism
A technology of multi-task learning and relation extraction, applied in the field of natural language relation extraction based on multi-task learning mechanism, which can solve the problems of inability to effectively deal with long-distance dependencies of sequences, accumulated errors, and obstacles to the effect of relation extraction tasks.
- Summary
- Abstract
- Description
- Claims
- Application Information
AI Technical Summary
Problems solved by technology
Method used
Image
Examples
Embodiment Construction
[0021] The present invention will be further described in detail in conjunction with the following specific embodiments and accompanying drawings. The process, conditions, experimental methods, etc. for implementing the present invention, except for the content specifically mentioned below, are common knowledge and common knowledge in this field, and the present invention has no special limitation content.
[0022] The present invention proposes a method for extracting natural language relations based on a multi-task learning mechanism, which is specifically divided into three parts, such as figure 2 Shown:
[0023] Input layer: mainly used to process input data. The input layer is similar to the input layer of the single-task model. It also first divides the sentence or sentence pair through WordPiece to obtain the subword sequence. However, unlike the single-task model, in order to avoid the problem of unbalanced multi-task data set size, the training samples of each auxi...
PUM
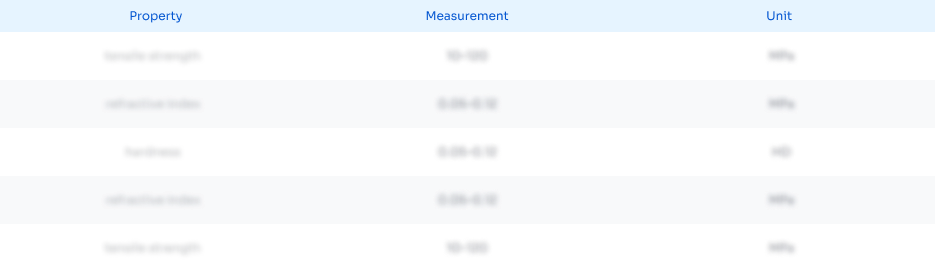
Abstract
Description
Claims
Application Information
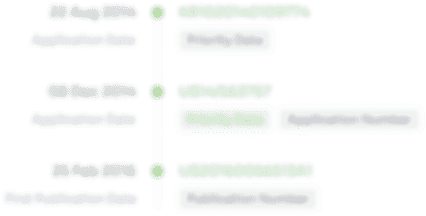
- R&D Engineer
- R&D Manager
- IP Professional
- Industry Leading Data Capabilities
- Powerful AI technology
- Patent DNA Extraction
Browse by: Latest US Patents, China's latest patents, Technical Efficacy Thesaurus, Application Domain, Technology Topic, Popular Technical Reports.
© 2024 PatSnap. All rights reserved.Legal|Privacy policy|Modern Slavery Act Transparency Statement|Sitemap|About US| Contact US: help@patsnap.com