Total electron content prediction method for global ionosphere based on deep learning framework
A technology of total electron content and deep learning, which is applied in the field of global ionospheric total electron content prediction, can solve the problems affecting the signal reception and application performance of navigation communication, the deterioration of the availability of navigation communication system, etc., so as to improve the prediction accuracy and improve the prediction accuracy Effect
- Summary
- Abstract
- Description
- Claims
- Application Information
AI Technical Summary
Problems solved by technology
Method used
Image
Examples
Embodiment Construction
[0018] The present invention will be described in detail below with reference to the accompanying drawings and specific embodiments. It should be pointed out that the described embodiments are only intended to facilitate understanding of the present invention and do not serve as any limitation.
[0019] The present invention proposes a method for predicting the total electron content of the global ionosphere based on a deep learning framework, which describes in detail the relationship between the total electron content of the ionosphere and influencing factors such as solar radiation, geomagnetic disturbance index, and upper atmosphere proportion components The prediction of the total electron content of the ionosphere is realized by constructing an optimized training model, and the prediction error is within the expected range.
[0020] Such as figure 1 Shown, a kind of global ionospheric total electron content prediction method based on deep learning framework of the presen...
PUM
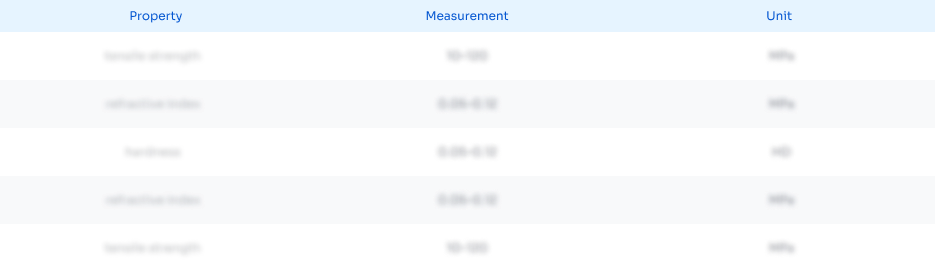
Abstract
Description
Claims
Application Information
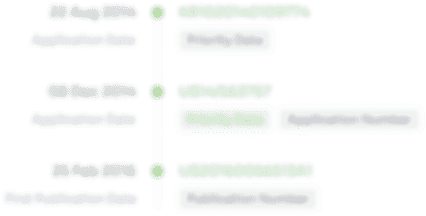
- R&D Engineer
- R&D Manager
- IP Professional
- Industry Leading Data Capabilities
- Powerful AI technology
- Patent DNA Extraction
Browse by: Latest US Patents, China's latest patents, Technical Efficacy Thesaurus, Application Domain, Technology Topic.
© 2024 PatSnap. All rights reserved.Legal|Privacy policy|Modern Slavery Act Transparency Statement|Sitemap