Systems and methods for depth estimation via affinity learned with convolutional spatial propagation networks
A space propagation and depth technology, applied in neural learning methods, biological neural network models, calculations, etc., can solve the problem of predicting the blurred depth
- Summary
- Abstract
- Description
- Claims
- Application Information
AI Technical Summary
Problems solved by technology
Method used
Image
Examples
Embodiment approach
[0046] Embodiments can be viewed as an anisotropic diffusion process that learns a diffusion tensor directly from a given image by a deep CNN to guide the refinement of the output.
[0047] 1. Convolutional Spatial Propagation Network
[0048] Given a depth map output from the network and image One task is to update the depth map to a new depth map D within N iteration steps n , which firstly reveals more details of the image and secondly improves the pixel-wise depth estimation results.
[0049] Figure 2B An update operation propagation process using CSPN is shown according to various embodiments of the present disclosure. Formally, without loss of generality, we can embed the depth map Do into some hidden space For each time step t, the convolutional transformation function with kernel size k can be written as:
[0050]
[0051] where κ i,j (0,0)=1-∑ a,b,a,b≠0 kappa i,j (a, b),
[0052]
[0053] Among them, the transformation kernel is spatially depen...
PUM
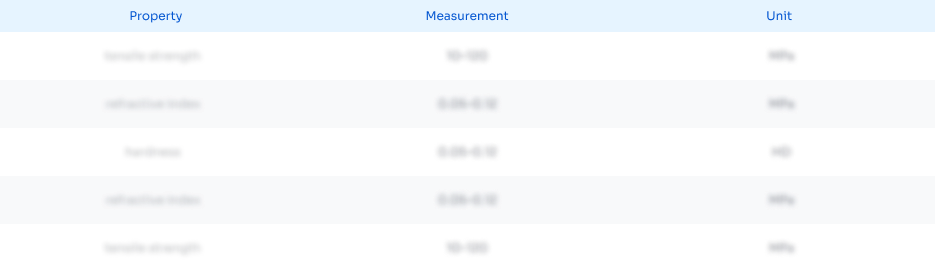
Abstract
Description
Claims
Application Information
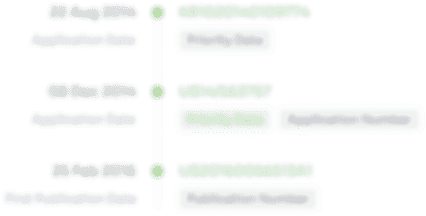
- R&D Engineer
- R&D Manager
- IP Professional
- Industry Leading Data Capabilities
- Powerful AI technology
- Patent DNA Extraction
Browse by: Latest US Patents, China's latest patents, Technical Efficacy Thesaurus, Application Domain, Technology Topic, Popular Technical Reports.
© 2024 PatSnap. All rights reserved.Legal|Privacy policy|Modern Slavery Act Transparency Statement|Sitemap|About US| Contact US: help@patsnap.com