Object pose estimation method based on self-supervised learning and template matching
A technology of template matching and supervised learning, applied in the field of computer vision, can solve problems such as difficulty in application promotion, difficulty in sample labeling, and lack of samples of the true value of the six-degree-of-freedom pose, so as to ensure high efficiency, avoid insufficient samples, and save difficulty and cost effects
- Summary
- Abstract
- Description
- Claims
- Application Information
AI Technical Summary
Problems solved by technology
Method used
Image
Examples
Embodiment Construction
[0061] The present invention will be described in detail below in conjunction with the accompanying drawings and specific embodiments. This embodiment is carried out on the premise of the technical solution of the present invention, and detailed implementation and specific operation process are given, but the protection scope of the present invention is not limited to the following embodiments.
[0062] An object pose estimation method based on self-supervised learning and template matching, including:
[0063] S1: Use a calibrated consumer-grade depth camera to collect the color image and depth image of the target object, and the color image and depth image are cropped by a convolutional neural network to obtain the corresponding color candidate image and depth candidate image;
[0064] S2: The color candidate map and the depth candidate map are segmented by a trained self-supervised codec to obtain a color segment map and a depth segment map;
[0065] S3: Use the color segm...
PUM
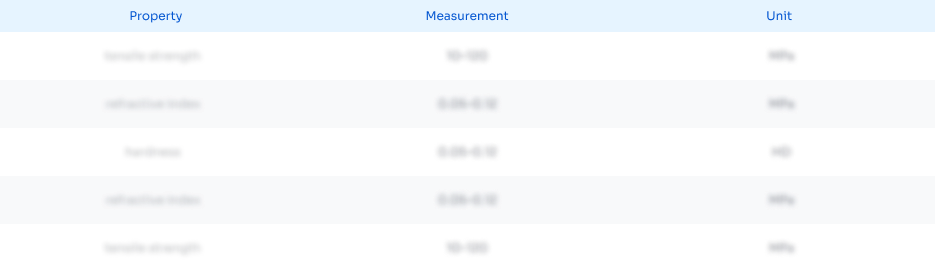
Abstract
Description
Claims
Application Information
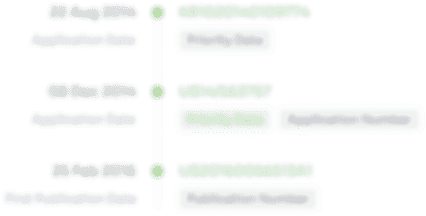
- R&D Engineer
- R&D Manager
- IP Professional
- Industry Leading Data Capabilities
- Powerful AI technology
- Patent DNA Extraction
Browse by: Latest US Patents, China's latest patents, Technical Efficacy Thesaurus, Application Domain, Technology Topic, Popular Technical Reports.
© 2024 PatSnap. All rights reserved.Legal|Privacy policy|Modern Slavery Act Transparency Statement|Sitemap|About US| Contact US: help@patsnap.com