Novel moving object destination prediction algorithm
A technology for moving objects and prediction algorithms, applied in the intersection of statistics and computer information science, can solve the problem of high computational cost, and achieve the effect of overcoming the problem of data sparseness, solving the problem of data sparseness and long-term dependence, and solving long-term dependence.
- Summary
- Abstract
- Description
- Claims
- Application Information
AI Technical Summary
Problems solved by technology
Method used
Image
Examples
Embodiment Construction
[0015] Below in conjunction with accompanying drawing, the present invention will be further described.
[0016] The invention is based on figure 1 The flow chart of , solves the problem of data sparsity and long-term dependence, and accurately predicts the trajectory destination while preventing the loss of effective information. First, the historical trajectory data and the trajectory data to be predicted are segmented, and the improved minimum description length (IMDL) method is used for processing, which preserves the characteristics of the original trajectory to a large extent, and at the same time segmented data for each trajectory. Trajectory segmentation solves the problem of data sparseness; then, using embedded technology, feature vectors are extracted, and the trajectory sequence is converted into an embedded vector sequence as the input of the prediction model. Through a part of the data set, the EP-LSTM model is first trained. When the training reaches a certain ...
PUM
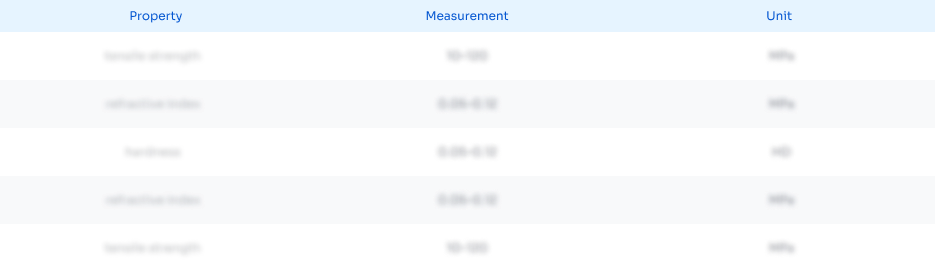
Abstract
Description
Claims
Application Information
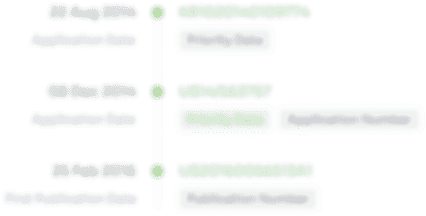
- R&D Engineer
- R&D Manager
- IP Professional
- Industry Leading Data Capabilities
- Powerful AI technology
- Patent DNA Extraction
Browse by: Latest US Patents, China's latest patents, Technical Efficacy Thesaurus, Application Domain, Technology Topic, Popular Technical Reports.
© 2024 PatSnap. All rights reserved.Legal|Privacy policy|Modern Slavery Act Transparency Statement|Sitemap|About US| Contact US: help@patsnap.com