Blind calibration method for data drift of distributed wireless sensor network
A wireless sensor and distributed data technology, applied in the field of communication, can solve the problems of high node energy consumption, general blind calibration method, and many application environment restrictions, so as to achieve good capture of signal characteristics, avoid data instability, and network expansion. strong effect
- Summary
- Abstract
- Description
- Claims
- Application Information
AI Technical Summary
Problems solved by technology
Method used
Image
Examples
Embodiment 1
[0029] Example 1: As the scale of WSN increases, the accuracy of collected data is also increasingly required. However, when the sensor collects data, due to its own hardware, there is a deviation between the actual signal and the measured signal, and data drift occurs. Calibration of data drift is necessary because drift may invalidate directly measured data. Usually, there are a large number of sensors in the network, and they are deployed in hard-to-reach locations. Manual calibration is very difficult. The centralized blind calibration method is prone to failure due to node failure. At the same time, network data needs to be collected in the server. Heavy, often many practical environments cannot meet its application.
[0030]In view of the above status quo, the present invention proposes a blind calibration method for distributed wireless sensor network data drift through research and innovation, see figure 1 , including the following steps:
[0031] Step 1 Determine a ...
Embodiment 2
[0043] Distributed wireless sensor network data drift blind calibration method is the same as embodiment 1, the assumed drift amount d described in step 1 and step 2 of the present invention i,t and additive noise v i,t Fits a Gaussian model, specifically:
[0044] additive noise v i,t The Gaussian model is as follows:
[0045]
[0046] in, Expressed as a mean of 0 and a variance of The additive noise Gaussian distribution of , N represents the Gaussian distribution;
[0047] Drift d i,t The Gaussian model is as follows:
[0048] d i,0 =μ i +β
[0049] d i,t = d i,t-1 +δ i,t
[0050] Among them, d i,0 Indicates the initial value of sensor i drift, expressed by μ i and β, where μ i is the variance of A Gaussian model with a mean of 0, β is the variance A Gaussian model with a mean of zero, or μ i for and variance Correlated Gaussian model, beta representation and variance The associated Gaussian model, δ i,t Indicates the drift increment of se...
Embodiment 3
[0053] The distributed wireless sensor network data drift blind calibration method is the same as the embodiment 1-2, and the parameters of the distributed data drift blind calibration model are trained using the training data described in step 3 of the present invention, specifically: the training data is 1×T The vector of , during model parameter training, according to the time sequence, intercept T p A data block with a length of time is used as an input for the parameter training of the distributed data drift blind calibration model, and the data block is cyclically intercepted in the same way to train the model parameters until the distributed wireless sensor network data drift blind calibration model parameters meet the training end condition, Usually the end condition is to reach the training round or meet the calibration accuracy requirement.
[0054] The present invention sets the length as T p The data block is used as the input of the distributed wireless sensor ne...
PUM
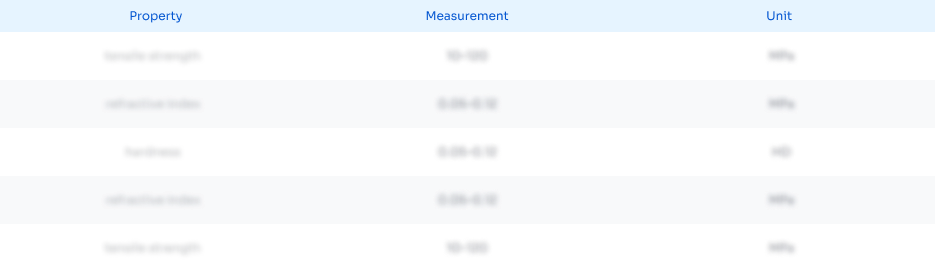
Abstract
Description
Claims
Application Information
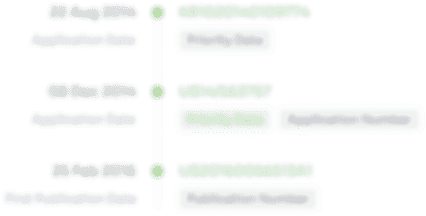
- R&D Engineer
- R&D Manager
- IP Professional
- Industry Leading Data Capabilities
- Powerful AI technology
- Patent DNA Extraction
Browse by: Latest US Patents, China's latest patents, Technical Efficacy Thesaurus, Application Domain, Technology Topic.
© 2024 PatSnap. All rights reserved.Legal|Privacy policy|Modern Slavery Act Transparency Statement|Sitemap