Double-window concept drift detection method based on sample distribution statistical test
A technology for statistical testing and sample distribution, applied in the field of machine learning, which can solve problems such as drift misjudgment, drift omission, and increase the update burden of the learner.
- Summary
- Abstract
- Description
- Claims
- Application Information
AI Technical Summary
Problems solved by technology
Method used
Image
Examples
Embodiment Construction
[0067] In order to verify the performance of this method, this paper selects the cement strength benchmark data set for testing. The data comes from the learning team of Prof. I-Cheng Yeh and can be obtained by visiting UCI (https: / / archive.ics.uci.edu / ). The data set contains a total of 1030 samples. The input variables are the main factors that directly or indirectly affect the compressive strength of cement, namely cement (Cement), blast furnace slag (Blast Furnace Slag), fly ash (Fly Ash), water (Water) , Superplasticizer, Coarse Aggregate, Fine Aggregate, Age, and the output is Concrete compressive strength.
[0068] Firstly, the data set is divided into two sub-data sets, which respectively contain the first 500 groups and the last 500 groups of data in the original data set, and then the two sub-data sets are divided into five equal intervals, each containing 100 groups of data. In this paper, the first data in the first sub-dataset is used as the training set for model...
PUM
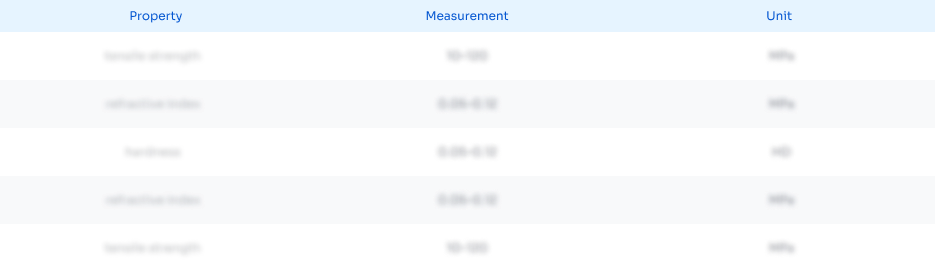
Abstract
Description
Claims
Application Information
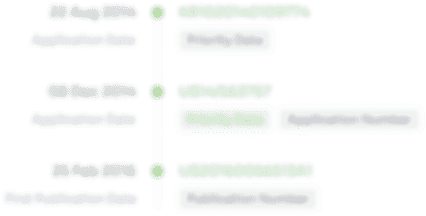
- Generate Ideas
- Intellectual Property
- Life Sciences
- Materials
- Tech Scout
- Unparalleled Data Quality
- Higher Quality Content
- 60% Fewer Hallucinations
Browse by: Latest US Patents, China's latest patents, Technical Efficacy Thesaurus, Application Domain, Technology Topic, Popular Technical Reports.
© 2025 PatSnap. All rights reserved.Legal|Privacy policy|Modern Slavery Act Transparency Statement|Sitemap|About US| Contact US: help@patsnap.com