Training method combining face recognition data equalization and enhancement
A face recognition and training method technology, applied in the field of image classification based on deep learning, can solve the problems of data and real scene differences, data imbalance, etc., and achieve low loss convergence value, high balance, and fast training convergence Effect
- Summary
- Abstract
- Description
- Claims
- Application Information
AI Technical Summary
Problems solved by technology
Method used
Image
Examples
Embodiment 1
[0043] A training method combining face recognition data equalization and enhancement, such as figure 1 shown, including the following steps:
[0044] S1. Generate a training pre-model using the photos contained in all the cluster label ids of the dataset;
[0045] S2. Screen out the enhanced sample picture bases in all clustering label ids of the data set; the enhanced sample picture bases are pictures whose quality meets certain conditions in each clustering label id;
[0046] S3. Perform offline enhancement on the enhanced sample image base, and filter out the similarity between each cluster label id in the enhanced sample image base and the cluster center of the cluster label id after offline enhancement in the similarity interval (a, b) n sample pictures between;
[0047] S4. Design a data equalization method to perform data equalization on the sample image;
[0048] S5. Perform online enhancement on the sample image after data equalization.
[0049] Working principle...
Embodiment 2
[0051] In order to realize the present invention better, further, combine figure 2 As shown, the S2 specifically includes the following steps:
[0052] S2.1. Use the pre-model generated by S1 to determine the class center of each class of cluster label id of the data set;
[0053] S2.2. According to the cluster center determined by the cluster label id of each category, select the pictures whose similarity with the cluster center is higher than the parameter t in the corresponding cluster label id.
[0054]Working principle: The class center of each cluster label id is determined through the pre-training model. For example, the glint dataset has 180,000 cluster label ids, that is, a matrix of 180000×512 is generated and saved. The calculation formula of each class center is as follows:
[0055] emb_i = model(img_i)
[0056] mid_emb = (emb_0 + emb_1 + ....emb_n) / n
[0057] mid_emb.shape: 1×512;
[0058] After the class center of each cluster label id is determined throug...
Embodiment 3
[0061] In order to better realize the present invention, further, the parameter t can be adjusted according to the size of the data set and actual requirements.
[0062] Working principle: Through the similarity parameter t, the pictures similar to the class center in the data set can be screened out, because the offline enhancement belongs to the category of large-scale enhancement, only the data near the class center is selected for selection, and the similarity parameter t is set for screening , can remove some outlayers and some data at the edge of the class; after many experiments in the present invention, the value of the parameter t is selected to be 0.65, but if the selected data sets are different, and the requirements for the accuracy of the experiment are different, The specific value of the similarity parameter t can be adjusted up and down independently.
[0063] Other parts of this embodiment are the same as those of Embodiment 1-2 above, so they will not be repe...
PUM
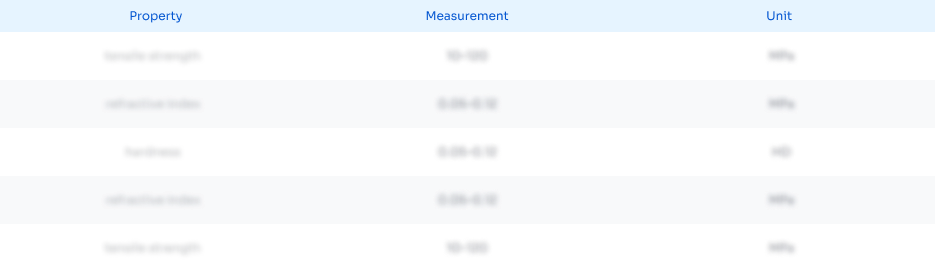
Abstract
Description
Claims
Application Information
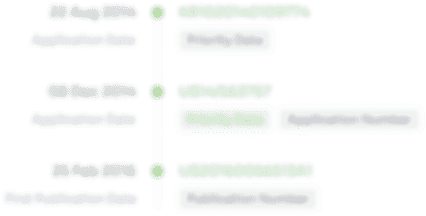
- R&D Engineer
- R&D Manager
- IP Professional
- Industry Leading Data Capabilities
- Powerful AI technology
- Patent DNA Extraction
Browse by: Latest US Patents, China's latest patents, Technical Efficacy Thesaurus, Application Domain, Technology Topic, Popular Technical Reports.
© 2024 PatSnap. All rights reserved.Legal|Privacy policy|Modern Slavery Act Transparency Statement|Sitemap|About US| Contact US: help@patsnap.com