Character interaction-oriented network weight generation few-sample image classification method
A technology of sample images and classification methods, applied in biological neural network models, instruments, character and pattern recognition, etc., can solve the problems of poor model generalization ability, easy overfitting, small image size, etc., to improve image classification ability , Solve the uneven distribution of images and reduce the effect of distribution differences
- Summary
- Abstract
- Description
- Claims
- Application Information
AI Technical Summary
Problems solved by technology
Method used
Image
Examples
Embodiment Construction
[0027] In the following, a method of network weight generation oriented to character interaction and few-sample image classification method of the present invention will be described in detail with reference to the embodiments and the accompanying drawings.
[0028] Such as figure 1 As shown, a kind of character interaction-oriented network weight generation few-sample image classification method of the present invention comprises the following steps:
[0029] 1) Divide the image data into a meta-training set and a meta-testing set; divide the meta-training set and meta-testing set into a support set and a query set respectively, and extract a set amount of samples from each category in the support set to form a small sample task ;
[0030] 2) Set the support set to contain w image categories, and each category is given by s quadruples Defined data, where s is an integer greater than or equal to 0, (figure 1 Take w=5, s=1 as an example) x i ∈R p is the i-th image visual f...
PUM
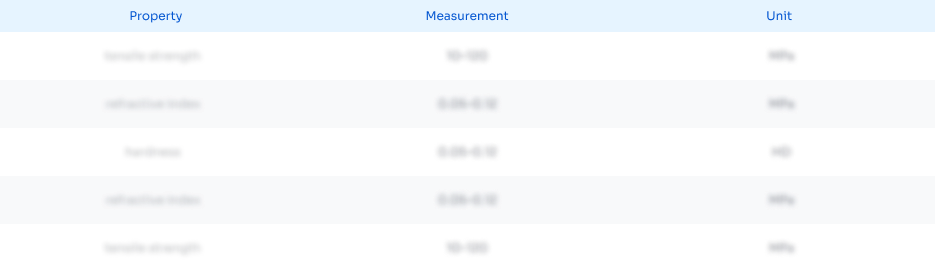
Abstract
Description
Claims
Application Information
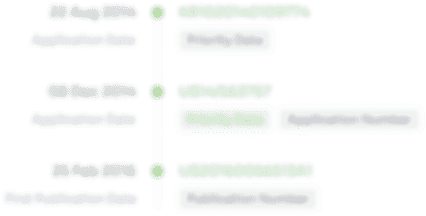
- R&D Engineer
- R&D Manager
- IP Professional
- Industry Leading Data Capabilities
- Powerful AI technology
- Patent DNA Extraction
Browse by: Latest US Patents, China's latest patents, Technical Efficacy Thesaurus, Application Domain, Technology Topic, Popular Technical Reports.
© 2024 PatSnap. All rights reserved.Legal|Privacy policy|Modern Slavery Act Transparency Statement|Sitemap|About US| Contact US: help@patsnap.com