Track data anonymity removing method based on deep learning
A technology of trajectory data and deep learning, applied in neural learning methods, digital data protection, structured data retrieval, etc., can solve problems such as manual intervention, insufficient research, and neglect of impact, and achieve the effect of performance improvement
- Summary
- Abstract
- Description
- Claims
- Application Information
AI Technical Summary
Problems solved by technology
Method used
Image
Examples
Embodiment Construction
[0026] In order to facilitate those of ordinary skill in the art to understand and implement the present invention, the present invention will be described in further detail below in conjunction with the accompanying drawings and embodiments. It should be understood that the implementation examples described here are only used to illustrate and explain the present invention, and are not intended to limit this invention.
[0027] In the field of deep learning, the emergence of ResNet has solved the problem of gradient dispersion very well, so that deeper networks can be trained better. The network of the L layer is formed by the network of the L-1 layer through the H (including Conv, BN, ReLU , Pooling) transformation, on this basis, it is directly connected to the network of the previous layer, so that the gradient can be better propagated. The basic idea of DenseNet proposed by "DenselyConnected Convolutional Networks" is consistent with ResNet, but it establishes a dense c...
PUM
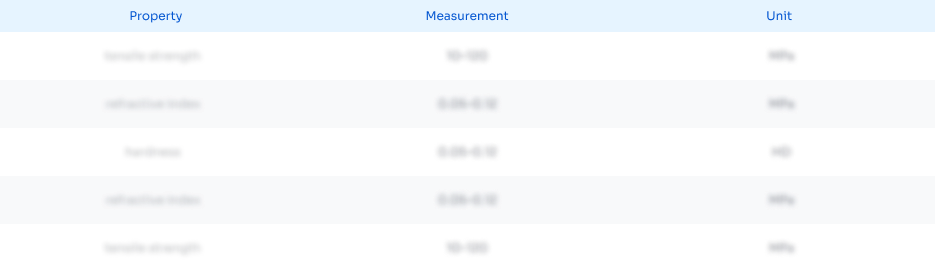
Abstract
Description
Claims
Application Information
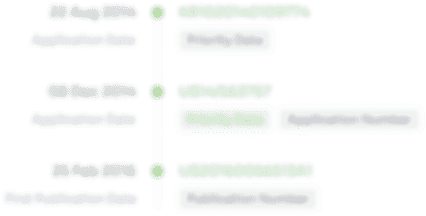
- R&D Engineer
- R&D Manager
- IP Professional
- Industry Leading Data Capabilities
- Powerful AI technology
- Patent DNA Extraction
Browse by: Latest US Patents, China's latest patents, Technical Efficacy Thesaurus, Application Domain, Technology Topic, Popular Technical Reports.
© 2024 PatSnap. All rights reserved.Legal|Privacy policy|Modern Slavery Act Transparency Statement|Sitemap|About US| Contact US: help@patsnap.com