Heat exchanger dirt growth prediction method based on PCA-GA-RBF
A prediction method and heat exchanger technology, applied in prediction, neural learning methods, instruments, etc., can solve problems such as slow response time and low precision
- Summary
- Abstract
- Description
- Claims
- Application Information
AI Technical Summary
Problems solved by technology
Method used
Image
Examples
Embodiment Construction
[0046] In order to better illustrate the application effect of the present invention, an application example of the method is now described.
[0047] refer to Figure 1 to Figure 5 , a PCA-GA-RBF-based prediction method for fouling growth in heat exchangers, including the following steps:
[0048]Step 1. Use the historical data of flow rate, temperature, pressure, fouling thermal resistance and physical parameters collected in petrochemical production to perform dimensionality reduction processing on the collected data through principal component analysis (PCA). Factors to reflect the complex internal laws that affect the formation of fouling, recombine a new set of unrelated data sets, and then construct the best heat exchanger fouling training samples;
[0049] 1.1) Assume that the original data set W has n×m dimensional data;
[0050]
[0051] 1.2) The original data is standardized, in order to eliminate the interference of dimensions, the following formula is used in ...
PUM
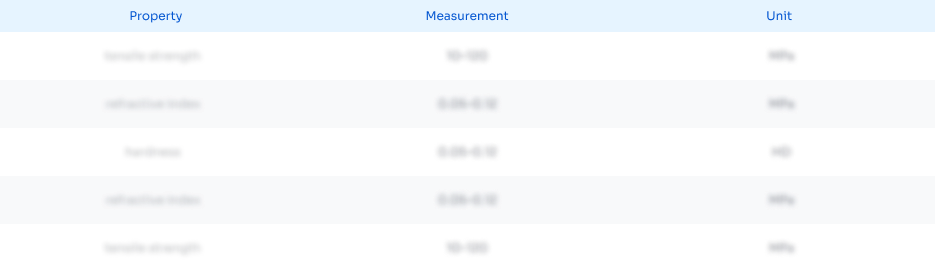
Abstract
Description
Claims
Application Information
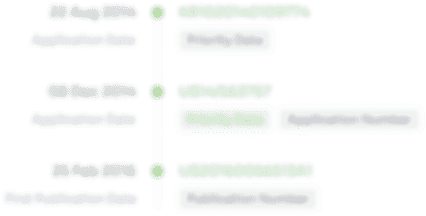
- R&D Engineer
- R&D Manager
- IP Professional
- Industry Leading Data Capabilities
- Powerful AI technology
- Patent DNA Extraction
Browse by: Latest US Patents, China's latest patents, Technical Efficacy Thesaurus, Application Domain, Technology Topic, Popular Technical Reports.
© 2024 PatSnap. All rights reserved.Legal|Privacy policy|Modern Slavery Act Transparency Statement|Sitemap|About US| Contact US: help@patsnap.com