Neural structure corresponding learning cross-domain emotion classification method for improving feature selection
A feature selection and emotion classification technology, applied in special data processing applications, instruments, electrical digital data processing, etc., can solve the feature redundancy without considering the importance of pivot feature text, affect the result of feature migration, and insufficient pivot features Reasonable and other issues to achieve the effect of reducing noise interference, improving accuracy, and reducing inter-domain differences
- Summary
- Abstract
- Description
- Claims
- Application Information
AI Technical Summary
Problems solved by technology
Method used
Image
Examples
Embodiment 1
[0035] Embodiment 1: as Figure 1-5 As shown, the neural structure of improved feature selection corresponds to learning a cross-domain emotion classification method, and the specific steps of the classification method are as follows:
[0036] Step1, using the Amazon product review dataset. Select two different domains as source domain and target domain data respectively. For the dataset source domain D s A small number of labeled samples of and the source domain D s , target domain D t A large number of unlabeled samples are used for text preprocessing to remove useless information and reduce noise interference. Use the parse tree function ElmentTree under the toolkit xml.tree to extract the Internet label corpus Comment sentences between;
[0037] Step2. Perform morphological restoration of the text, eliminate redundant features, and vectorize the text to obtain the initial features of the text; and use the chi-square test feature selection method to filter out pivot ...
Embodiment 2
[0052] Embodiment 2: as Figure 1-5 As shown, the neural structure of improved feature selection corresponds to learning a cross-domain emotion classification method, and the specific steps of the classification method are as follows:
[0053] Step1, using the Amazon product review dataset. The data statistics table is shown in Table 1, and two different fields are selected as the source domain D s and target domain D t ; Since the data set is Internet tag data, use the parse tree function Element.Tree under the xml.etree tree to extract the Internet tag corpus Between the comment sentences, the text content of the source domain and the target domain are obtained. will come from source domain D s A small number of labeled samples of and the source domain D s , target domain D t A large number of unlabeled samples are processed by removing stop words to reduce noise interference.
[0054] Table 1 Amazon product review statistics table
[0055] data set posi...
PUM
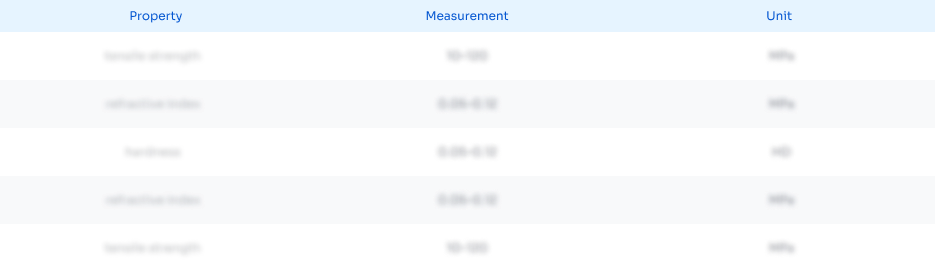
Abstract
Description
Claims
Application Information
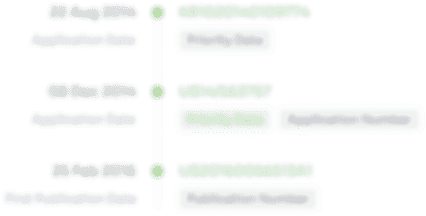
- Generate Ideas
- Intellectual Property
- Life Sciences
- Materials
- Tech Scout
- Unparalleled Data Quality
- Higher Quality Content
- 60% Fewer Hallucinations
Browse by: Latest US Patents, China's latest patents, Technical Efficacy Thesaurus, Application Domain, Technology Topic, Popular Technical Reports.
© 2025 PatSnap. All rights reserved.Legal|Privacy policy|Modern Slavery Act Transparency Statement|Sitemap|About US| Contact US: help@patsnap.com