Micro-seismic signal identification method based on quasi-optimal Gaussian kernel multi-classification support vector machine
A support vector machine and signal recognition technology, applied in seismic signal processing, character and pattern recognition, seismology, etc., can solve problems such as excessive data volume, unbalanced samples, and low signal-to-noise ratio
- Summary
- Abstract
- Description
- Claims
- Application Information
AI Technical Summary
Problems solved by technology
Method used
Image
Examples
Embodiment 1
[0100] The microseismic monitoring system of a coal mine roadway is composed of 20 microseismic detection probes. The time window for microseismic data processing is set to 4 hours. Each microseismic detection probe contains 10240 microseismic signal sampling values in this time window. A set of microseismic signals. According to the sampling time range, a total of 235 groups of microseismic signals were obtained.
[0101] Using the method described in step 2, calculate the energy values of 235 groups of microseismic signals to determine their strength. Among them, the energy calculation values of 20 groups of microseismic signals are shown in Table 1. Among the 235 groups of microseismic signals, a total of 200 were marked as weak microseismic signals, and a total of 35 were marked as strong microseismic signals.
[0102] Table 1 Energy distribution of microseismic signals
[0103] serial number 1 2 3 4 5 6 7 Energy / J 2.42×10 7
7.98×10 6
...
PUM
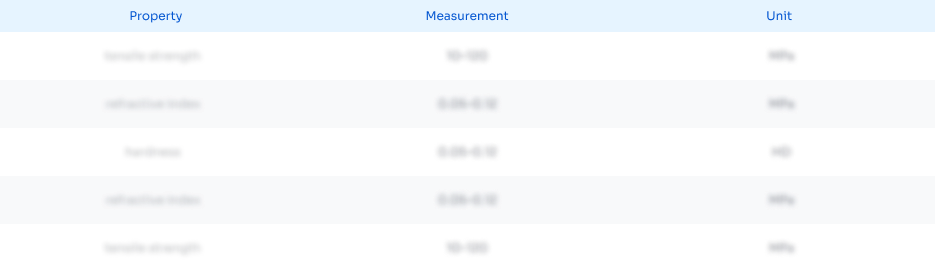
Abstract
Description
Claims
Application Information
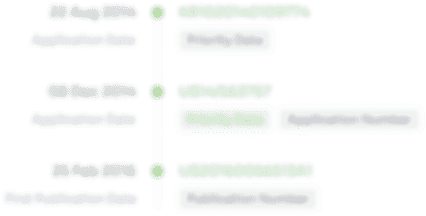
- R&D Engineer
- R&D Manager
- IP Professional
- Industry Leading Data Capabilities
- Powerful AI technology
- Patent DNA Extraction
Browse by: Latest US Patents, China's latest patents, Technical Efficacy Thesaurus, Application Domain, Technology Topic.
© 2024 PatSnap. All rights reserved.Legal|Privacy policy|Modern Slavery Act Transparency Statement|Sitemap