Fine-grained sentiment classification method
A sentiment classification, fine-grained technology, applied in neural learning methods, text database clustering/classification, biological neural network models, etc., can solve the problems of weakening network feature expression ability, poor performance, etc., to improve the discrimination accuracy, Improve accuracy and improve network performance
- Summary
- Abstract
- Description
- Claims
- Application Information
AI Technical Summary
Problems solved by technology
Method used
Image
Examples
Embodiment 1
[0082] Such as Figure 1 to Figure 3 As shown, a fine-grained sentiment classification method includes the following steps:
[0083] Step 1: the input sentence is preprocessed, and the preprocessed sentence is mapped to a low-dimensional dense word vector in the form of a table lookup;
[0084] Step 2: Input the word vector of the sentence, and the two-way LSTM network performs feature extraction on the word vector of the sentence to obtain the semantic feature information of the sentence
[0085] Step 3: Utilize the semantic feature information of the sentence and attention mechanism to extract feature information of target attributes Using the method of residual connection, the feature information of the target attribute Semantic feature information Perform information fusion to obtain feature information feature information Carry out position encoding to obtain memory information Use location information L o extended memory information Form the network me...
Embodiment 2
[0133] Such as Figure 2 to Figure 4 As shown, a fine-grained sentiment classification system includes:
[0134] Preprocessing layer 1, used to preprocess the input sentence;
[0135] The word vector layer 2 is used to map the preprocessed sentence into a low-dimensional dense word vector by means of a table lookup;
[0136]Bidirectional LSTM network layer 3, used for feature extraction of the word vector of the sentence, to obtain the semantic feature information of the sentence
[0137] Memory network layer 4, used to utilize the semantic feature information of the sentence and attention mechanism to extract feature information of target attributes Using the method of residual connection, the feature information of the target attribute Semantic feature information Perform information fusion to obtain feature information feature information Carry out position encoding to obtain memory information Use location information L o extended memory information For...
PUM
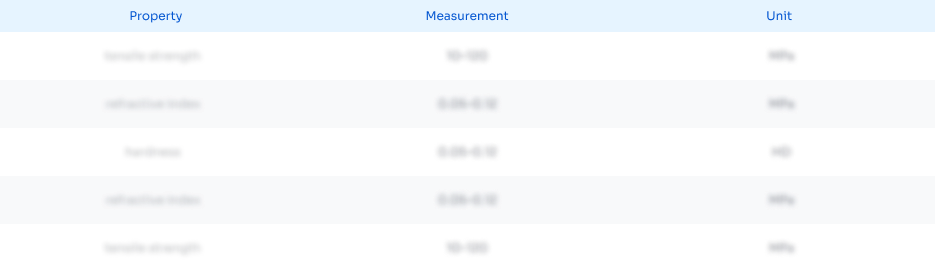
Abstract
Description
Claims
Application Information
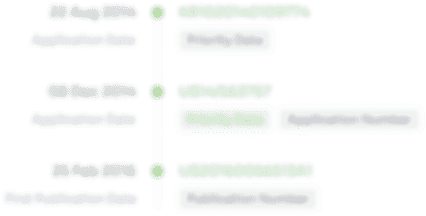
- R&D Engineer
- R&D Manager
- IP Professional
- Industry Leading Data Capabilities
- Powerful AI technology
- Patent DNA Extraction
Browse by: Latest US Patents, China's latest patents, Technical Efficacy Thesaurus, Application Domain, Technology Topic, Popular Technical Reports.
© 2024 PatSnap. All rights reserved.Legal|Privacy policy|Modern Slavery Act Transparency Statement|Sitemap|About US| Contact US: help@patsnap.com