Multi-target classification method based on vehicle-mounted millimeter-wave radar combined with svm and cnn
A millimeter wave radar and classification method technology, applied in the field of joint support vector machine and convolutional neural network target classification, can solve the problems of ignoring position information and poor classification effect, achieve strong generalization ability, avoid artificial feature extraction, improve The effect of accuracy
- Summary
- Abstract
- Description
- Claims
- Application Information
AI Technical Summary
Problems solved by technology
Method used
Image
Examples
Embodiment 1
[0054] Such as figure 1 As shown, based on the vehicle-mounted millimeter-wave radar joint SVM and CNN multi-target classification method, including: training phase and testing phase; the training phase includes the following steps:
[0055] Step 1. Obtain the intermediate frequency signal f(t) after the target echo signal is processed by the millimeter wave radar system, and calibrate the classification label for each obtained intermediate frequency signal f(t);
[0056] The millimeter-wave radar system is installed on the vehicle, and the millimeter-wave radar system includes a radar transmitter, a radar receiver and a mixer; the radar transmitter periodically transmits a chirp signal, and the radar receiver receives the echo scattered by the target. Wave signal, the mixer uses the chirp signal transmitted by the radar to mix the received echo signal to obtain the intermediate frequency signal f(t);
[0057] In the training phase, pedestrians, bicycles and cars are respecti...
Embodiment 2
[0095] Embodiment 1 constructs a sample set by acquiring the intermediate frequency signal f(t) processed by the millimeter wave radar system on the target echo signal. When the number of collected intermediate frequency signals f(t) of known target categories is insufficient, the intermediate frequency signal f(t) can be generated by means of simulation. Using signal simulation to generate intermediate frequency signals corresponding to echo signals of pedestrians, bicycles, and cars, you can refer to the Chinese invention patent application with application number 2019104895140.
[0096] In this embodiment, 40 IF signals of pedestrians, 80 IF signals of bicycles and 200 IF signals of automobiles are generated through signal simulation. Process each generated IF signal to generate multiple range-Doppler maps, and extract 5 range-Doppler maps at equal intervals from the multiple range-Doppler maps generated by each IF signal, totaling 1600, And calibrate the category label of...
PUM
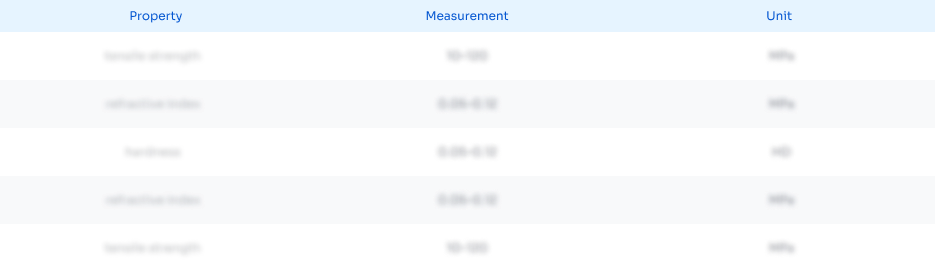
Abstract
Description
Claims
Application Information
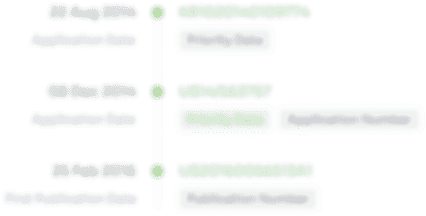
- R&D Engineer
- R&D Manager
- IP Professional
- Industry Leading Data Capabilities
- Powerful AI technology
- Patent DNA Extraction
Browse by: Latest US Patents, China's latest patents, Technical Efficacy Thesaurus, Application Domain, Technology Topic, Popular Technical Reports.
© 2024 PatSnap. All rights reserved.Legal|Privacy policy|Modern Slavery Act Transparency Statement|Sitemap|About US| Contact US: help@patsnap.com