Multi-class out-of-order workpiece robot grabbing pose estimation method based on deep learning
A technology of deep learning and pose estimation, applied in neural learning methods, instruments, calculations, etc., can solve the problems of long cycle, low efficiency, and limited expressive ability of complex functions, and achieve the effect of long cycle and low efficiency
- Summary
- Abstract
- Description
- Claims
- Application Information
AI Technical Summary
Problems solved by technology
Method used
Image
Examples
Embodiment Construction
[0034] The present invention will be further described below in conjunction with drawings and embodiments.
[0035] The training process of the deep learning network of the specific embodiment of the present invention is as follows:
[0036]The implemented system consists of three independent deep learning networks, which are respectively point cloud classification network, position generation network and attitude generation network. The point cloud classification network, position generation network and attitude generation network all adopt the same network structure, specifically including Connected random sampling layer, perceptual layer, pooling layer and the final multi-layer perceptron, the same perceptual layer is composed of multiple multi-layer perceptrons connected in parallel, each multi-layer perceptron in the perceptual layer shares / has the same parameters, random The sampling layer receives the input data for random sampling, and then inputs each set of randomly ...
PUM
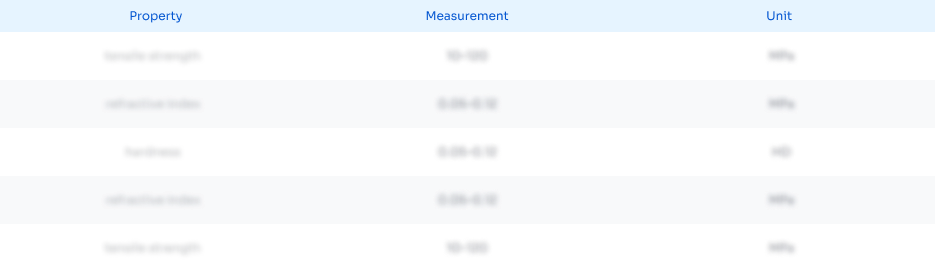
Abstract
Description
Claims
Application Information
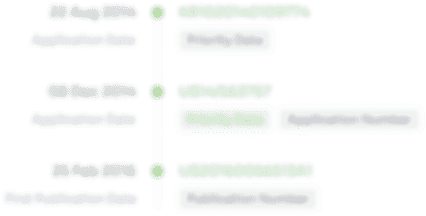
- R&D Engineer
- R&D Manager
- IP Professional
- Industry Leading Data Capabilities
- Powerful AI technology
- Patent DNA Extraction
Browse by: Latest US Patents, China's latest patents, Technical Efficacy Thesaurus, Application Domain, Technology Topic, Popular Technical Reports.
© 2024 PatSnap. All rights reserved.Legal|Privacy policy|Modern Slavery Act Transparency Statement|Sitemap|About US| Contact US: help@patsnap.com