A 4D-CBCT Imaging Method Based on Motion Compensated Learning
A 4D-CBCT, motion compensation technology, applied in the fields of radiological diagnosis instruments, image analysis, medical science, etc., can solve the problem of ineffective removal of star-stripe artifacts and noise, and achieve the improvement of details that are easy to lose and reduce. Additional radiation, improved artifact-heavy effects
- Summary
- Abstract
- Description
- Claims
- Application Information
AI Technical Summary
Problems solved by technology
Method used
Image
Examples
Embodiment 1
[0048] A flow chart of a 4D-CBCT imaging method based on motion compensation learning in this embodiment is as follows figure 1 As shown, the specific steps are as follows:
[0049] Step 1. Prepare the training data set required by the network.
[0050] Select high-quality 4D-CBCT training reconstruction images from the hospital image database where T is the total number of phases, V t p For the CBCT reconstruction image at phase t, when the intermediate phase reconstruction image is selected For label phase data, the rest of the phase reconstruction map V t p is the sample phase data; when the intermediate phase reconstruction map is selected For the sample phase data, the rest of the phase reconstruction map V t p is the tag phase data; t≠t 1 ;
[0051] Specifically, when using a specific training data set, for example, when radiotherapy is performed on the same patient, it is necessary to locate and track the tumor in real time, which requires high-quality 4D-C...
PUM
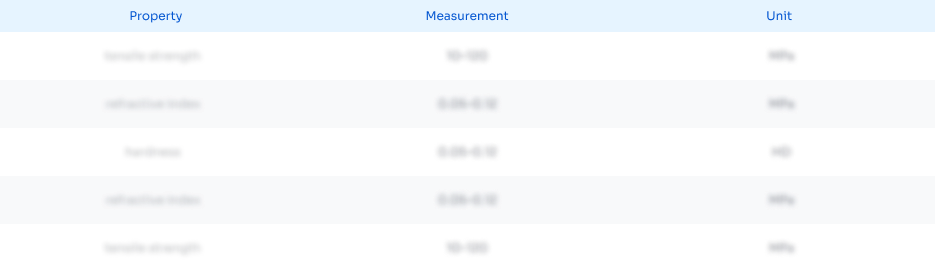
Abstract
Description
Claims
Application Information
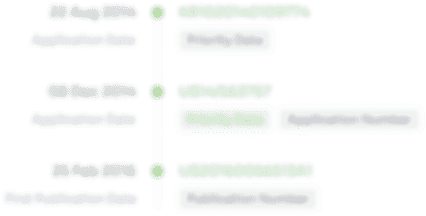
- R&D Engineer
- R&D Manager
- IP Professional
- Industry Leading Data Capabilities
- Powerful AI technology
- Patent DNA Extraction
Browse by: Latest US Patents, China's latest patents, Technical Efficacy Thesaurus, Application Domain, Technology Topic, Popular Technical Reports.
© 2024 PatSnap. All rights reserved.Legal|Privacy policy|Modern Slavery Act Transparency Statement|Sitemap|About US| Contact US: help@patsnap.com