Lane line detection method based on deep learning
A lane line detection, deep learning technology, applied in the direction of instruments, character and pattern recognition, computer parts, etc., can solve the problems of time-consuming detection accuracy and other problems, achieve the effect of overcoming time-consuming, accurate and rapid detection, and overcome the accuracy of detection low effect
- Summary
- Abstract
- Description
- Claims
- Application Information
AI Technical Summary
Problems solved by technology
Method used
Image
Examples
specific Embodiment approach 1
[0018] Specific implementation mode one: as figure 1 As shown, the lane line detection method based on deep learning described in this embodiment comprises the following steps:
[0019] Step 1. Randomly select M images from the TuSimple dataset, and mark the lane lines contained in the selected M images to obtain the marked image;
[0020] Step 2. Input the marked image obtained in step 1 into the fully convolutional neural network FCN8s, use the input image to train the fully convolutional neural network FCN8s, stop the training until the loss function value no longer decreases, and obtain the trained full convolution neural network FCN8s;
[0021] The network structure of FCN can be divided into FCN-32s, FCN-16s, FCN-8s according to finally returning to the multiple of the input image size, select FCN-8s among the present invention;
[0022] Step 3, input the image to be detected by the lane line into the fully convolutional neural network FCN8s trained in step 2, and obta...
specific Embodiment approach 2
[0037] Specific embodiment 2: The difference between this embodiment and specific embodiment 1 is that the loss function used in the step 2 is a weighted cross entropy loss function (Weighted Cross Entropy Loss), for any pixel in the image to be tested, The true category of the pixel is y (y=1 means that the pixel is a lane line point, otherwise the pixel point is a non-lane line point), and the probability that the pixel is predicted to be class y is p, then the pixel’s cross entropy loss value WCE (p,y) is:
[0038] WCE(p,y)=-α t log(p t )
[0039] in: alpha t Represents the weight coefficient;
[0040] Add the cross-entropy loss values of all pixels in the image to be tested to obtain the total cross-entropy loss value;
[0041] Stop training until the total cross-entropy loss value no longer decreases.
[0042] The difference between the loss function of this embodiment and the standard cross-entropy loss function is that there is an additional item α t parameter...
specific Embodiment approach 3
[0047] Embodiment 3: The difference between this embodiment and Embodiment 1 is that the loss function used in the second step is Focal Loss (Lin T Y, Goyal P, Girshick R, et al. Focal Loss for DenseObject Detection[J] .IEEE Transactions on Pattern Analysis&Machine Intelligence, 2017, PP(99):2999-3007), for any pixel in the image to be tested, the true category of the pixel is y, and the probability of the pixel predicted as category y is p, then the The loss value FL(p,y) of the pixel is:
[0048] FL(p,y)=-α t (1-p t ) γ log(p t )
[0049] in: alpha t and γ both represent weight coefficients;
[0050] Add the loss values of all pixels in the image to be tested to obtain the total loss value;
[0051] Stop training until the total loss value no longer decreases.
[0052] The loss function of this embodiment is multiplied on the basis of the weighted cross-entropy loss function by (1-p t ) γ , which can balance the difference between easy-to-classify sample points...
PUM
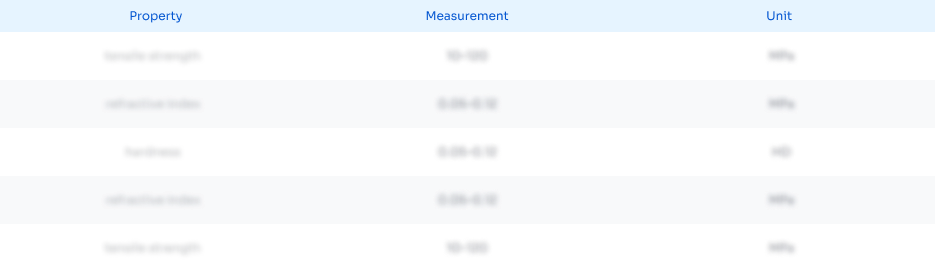
Abstract
Description
Claims
Application Information
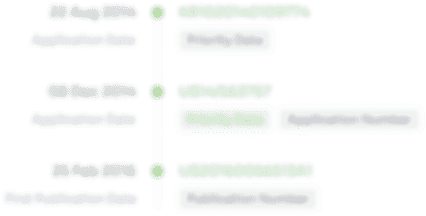
- R&D
- Intellectual Property
- Life Sciences
- Materials
- Tech Scout
- Unparalleled Data Quality
- Higher Quality Content
- 60% Fewer Hallucinations
Browse by: Latest US Patents, China's latest patents, Technical Efficacy Thesaurus, Application Domain, Technology Topic, Popular Technical Reports.
© 2025 PatSnap. All rights reserved.Legal|Privacy policy|Modern Slavery Act Transparency Statement|Sitemap|About US| Contact US: help@patsnap.com