Non-intrusive load decomposition and monitoring method based on difference
A differential and differential information technology, applied in the field of non-intrusive load decomposition and monitoring, can solve problems such as increasing the learning difficulty of neural networks, limiting decomposition accuracy, etc., achieving the effect of easy learning and training, reducing requirements, and improving usability
- Summary
- Abstract
- Description
- Claims
- Application Information
AI Technical Summary
Problems solved by technology
Method used
Image
Examples
Embodiment 1
[0066] This embodiment provides a non-intrusive load decomposition method based on differential input strategy and neural network, the flow chart is shown in the attached figure 1 , the specific processing flow includes the following steps:
[0067] S1. First obtain the total real-time power X of each electrical appliance on the master meter, and at the same time acquire the real-time power Y of the target electrical appliance (target load) at the corresponding time.
[0068] S2. Calculate the differential information of the total real-time power X on the master meter, that is, calculate the real-time variation of the total power, and obtain the real-time power variation sequence Xd.
[0069] S3, using the power change sequence Xd as the input of the neural network model, and using the real-time power Y at the time corresponding to the target load as the output of the neural network model to obtain a training sample set;
[0070] S4. Using the training sample set to train the...
Embodiment 2
[0107] Through the above description, we understand the implementation process of the non-intrusive load splitting method based on the differential input strategy and neural network of the present invention. This process can be realized by the non-intrusive load splitting device. Next, we will describe the interior of the non-intrusive load splitting device of the present invention Structure and function are introduced.
[0108] see Figure 5 , in the embodiment of the present invention, the non-intrusive load decomposition device 10 includes: an acquisition module 101 , a difference module 102 , a sliding module 103 and an analysis module 104 .
[0109] The acquiring module 101 is used to acquire the total real-time power X of each electrical appliance on the master meter, and at the same time acquire the real-time power Y of the target electrical appliance at a corresponding time.
[0110] The difference module 102 is used to calculate the difference information of the tota...
Embodiment 3
[0129] Figure 7 It is a schematic structural diagram of a non-intrusive load decomposition and inference device 100 based on a differential input strategy and a neural network according to Embodiment 3 of the present invention. The device 100 may have relatively large differences due to different configurations or performances, and may include one or more central processing units (central processing units, CPU) 1001 (for example, one or more processors) and memory 1002 . Wherein, the program for executing the non-intrusive load decomposition and inference method of the present invention can be stored in the memory 1002, and the central processing unit 1001 can be configured to communicate with the memory 1002 to execute the non-intrusive load decomposition and inference method on the device 100 A series of command operations.
[0130] Those skilled in the art should understand that the embodiments of the present invention may be provided as methods, systems, or computer prog...
PUM
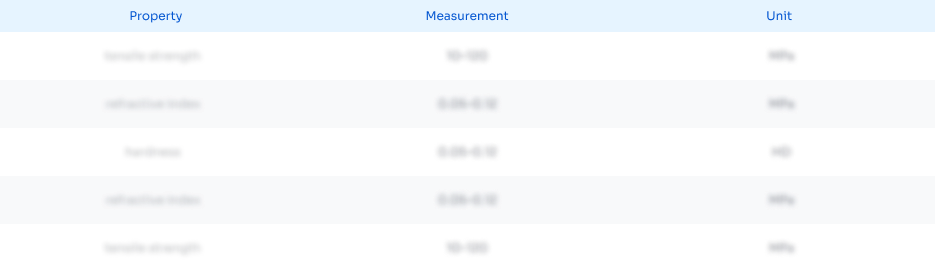
Abstract
Description
Claims
Application Information
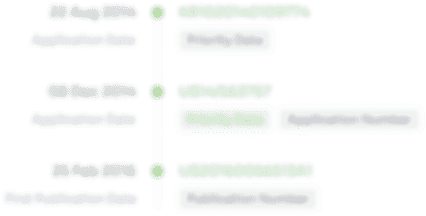
- R&D Engineer
- R&D Manager
- IP Professional
- Industry Leading Data Capabilities
- Powerful AI technology
- Patent DNA Extraction
Browse by: Latest US Patents, China's latest patents, Technical Efficacy Thesaurus, Application Domain, Technology Topic, Popular Technical Reports.
© 2024 PatSnap. All rights reserved.Legal|Privacy policy|Modern Slavery Act Transparency Statement|Sitemap|About US| Contact US: help@patsnap.com