Feature enhancement-based residual neural network and image deblocking effect method
A feature enhancement, neural network technology, applied in image communication, image enhancement, image analysis and other directions, can solve the problem of not fully extracting image feature information, hindering the improvement of network performance, simple network structure, etc., to enhance learning ability and Modeling capabilities, enhancement of overall features and dimensionality reduction, the effect of increasing network depth
- Summary
- Abstract
- Description
- Claims
- Application Information
AI Technical Summary
Problems solved by technology
Method used
Image
Examples
Embodiment Construction
[0040] The present invention will be further described below with reference to the accompanying drawings and in combination with preferred embodiments.
[0041] This embodiment provides an image deblocking method based on a feature-enhanced residual neural network, and its flow chart is as follows Figure 5 shown; including the following steps:
[0042] Step 1: Build a feature-enhanced residual neural network model;
[0043] The feature enhanced residual neural network model is as figure 1 As shown, it includes: 2 convolutional layers, 3 local residual units, 2 global feature enhancement units and 1 local feature enhancement unit, the 3 local residual units and 2 global feature enhancement units The rules of connecting a global feature enhancement unit among the local residual units are sequentially connected as a branch, and the local feature enhancement unit is used as another branch; the shallow features extracted by the input image through a convolutional layer are respe...
PUM
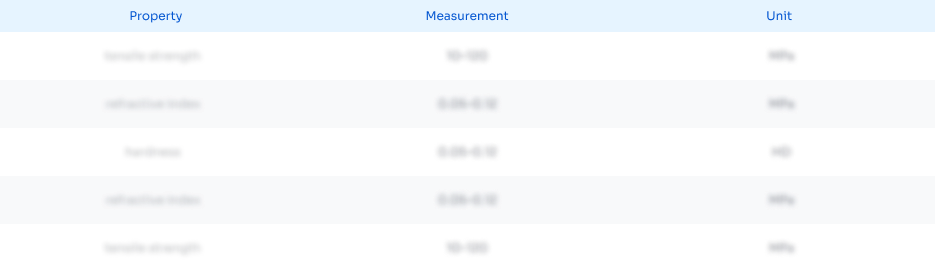
Abstract
Description
Claims
Application Information
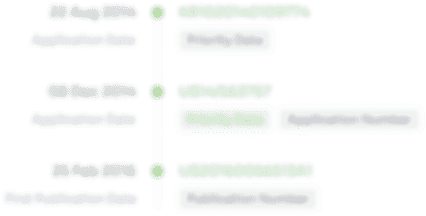
- R&D Engineer
- R&D Manager
- IP Professional
- Industry Leading Data Capabilities
- Powerful AI technology
- Patent DNA Extraction
Browse by: Latest US Patents, China's latest patents, Technical Efficacy Thesaurus, Application Domain, Technology Topic, Popular Technical Reports.
© 2024 PatSnap. All rights reserved.Legal|Privacy policy|Modern Slavery Act Transparency Statement|Sitemap|About US| Contact US: help@patsnap.com