Road scene defogging method based on conditional generative adversarial network
A condition generation and road technology, applied in the field of image processing, can solve the problems of rare, missing data, etc., achieve good robustness, good defogging effect, and reduce image processing time
- Summary
- Abstract
- Description
- Claims
- Application Information
AI Technical Summary
Problems solved by technology
Method used
Image
Examples
Embodiment
[0029] Below in conjunction with accompanying drawing, further describe the present invention by example, but do not limit the scope of the present invention in any way.
[0030] The present invention takes the RESIDE data set as an example, and the specific operations are as follows:
[0031] Step 1: Use the RESIDE dataset, which contains training sets and test sets;
[0032] Step 2: Send the foggy image x in the training set as input to the generator G to generate G(x), that is, the fog-free image
[0033] Step 3: Convert the generated fog-free image to Send it to the discriminator D to judge whether the picture is true or false;
[0034] Step 4: After 200 iterations, the optimal generation model is obtained;
[0035] Step 5: Apply the obtained optimal generation model to real foggy images for dehazing processing.
[0036] In this implementation, the generator is composed of three parts: encoder, converter and decoder; the encoder is composed of 3 layers of convolutio...
PUM
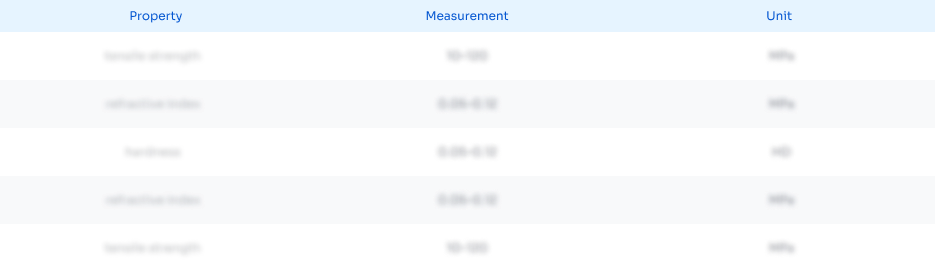
Abstract
Description
Claims
Application Information
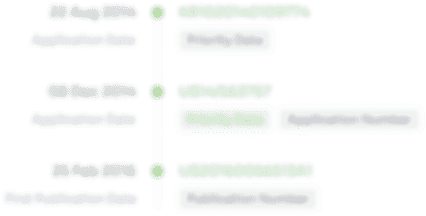
- R&D Engineer
- R&D Manager
- IP Professional
- Industry Leading Data Capabilities
- Powerful AI technology
- Patent DNA Extraction
Browse by: Latest US Patents, China's latest patents, Technical Efficacy Thesaurus, Application Domain, Technology Topic, Popular Technical Reports.
© 2024 PatSnap. All rights reserved.Legal|Privacy policy|Modern Slavery Act Transparency Statement|Sitemap|About US| Contact US: help@patsnap.com