Medicament module pharmacokinetic property and toxicity predicting method based on capsule network
A technology of pharmacokinetics and drug molecules, which is applied in the field of pharmacokinetic properties and toxicity prediction of drug molecules based on capsule network, can solve the large dependence of training set size, loss of original information of molecular fingerprints and molecular descriptors, Problems such as poor prediction and classification
- Summary
- Abstract
- Description
- Claims
- Application Information
AI Technical Summary
Problems solved by technology
Method used
Image
Examples
Embodiment 1
[0138] Predict the active compound of the potassium ion channel encoded by hERG (human ether-a-go-go-related gene), and use the convolution operation as a feature extractor to obtain the low-level features of the molecule. The implementation process is as follows:
[0139] In the first step, data related to hERG activity are collected. Data related to hERG were obtained from the ChEMBL open source database (https: / / www.ebi.ac.uk / chembl / ). ChEMBL is a well-known biological activity database established by the European Institute of Bioinformatics. Anyone can download this public database from the website, so it is widely used by cheminformatics researchers. The workflow for establishing the initial ChEMBL-hERG dataset is as follows:
[0140] 1) According to the ID number of hERG in the data (ChEMBL 240), 17,952 compounds that were tested for hERG activity were extracted;
[0141] 2) Compounds identified as "Nonstandard unit for type", "Outside typical range" (outside typical...
Embodiment 2
[0152] Still predicting the active compounds of the potassium ion channel encoded by hERG (human ether-a-go-go-related gene), a restricted Boltzmann machine was used as a feature extractor.
[0153] The first and second steps are the same as in Example 1.
[0154] In the third step, the prediction model of hERG active / inactive molecules based on the capsule network was established with the ChEMBL-hERG training set. Initialize network weights randomly using a truncated normal distribution and set stddev to 0.01. The feature extractor uses a restricted Boltzmann machine. The probability distribution of the energy function is used as the activation function. To reduce internal covariate shift, the input distribution of each layer is normalized to a standard Gaussian distribution using a batch normalization method. Adam method is used for network optimization. By monitoring multiple evaluation indicators (accuracy, specificity, sensitivity and Matthews correlation coefficient,...
Embodiment 1、2
[0162] The present embodiment 1, 2 adopts the following formula to carry out evaluation verification:
[0163]
[0164]
[0165]
[0166] Where Q represents the overall prediction accuracy of the prediction model, SE represents sensitivity, which refers to the proportion of positive / active compounds correctly predicted by the prediction model, and SP represents specificity, which refers to the proportion of negative / inactive compounds correctly predicted by the prediction model .
[0167] When convolution and restricted Boltzmann machine are used as feature extractors, the overall prediction accuracy of the test set is about 90%, indicating that the established model also has a good predictive ability for compounds independent of the training set.
PUM
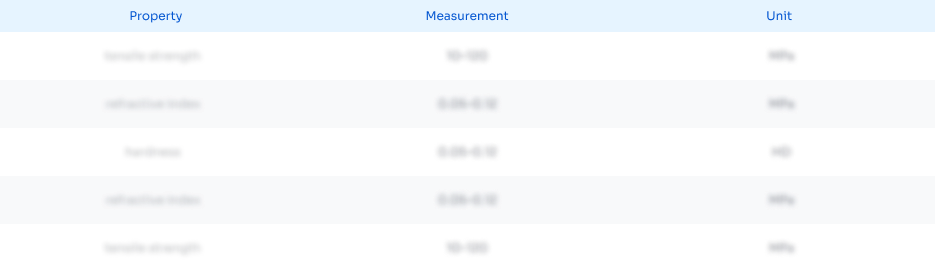
Abstract
Description
Claims
Application Information
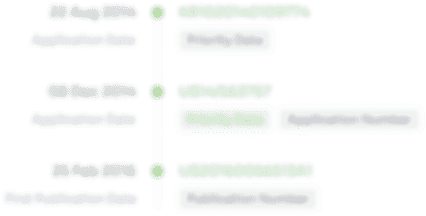
- R&D Engineer
- R&D Manager
- IP Professional
- Industry Leading Data Capabilities
- Powerful AI technology
- Patent DNA Extraction
Browse by: Latest US Patents, China's latest patents, Technical Efficacy Thesaurus, Application Domain, Technology Topic, Popular Technical Reports.
© 2024 PatSnap. All rights reserved.Legal|Privacy policy|Modern Slavery Act Transparency Statement|Sitemap|About US| Contact US: help@patsnap.com