A Soft Tissue Penetration Force Modeling Method Based on Segmented Artificial Neural Network
A technology of neural network and modeling method, applied in the direction of biological neural network model, neural learning method, neural architecture, etc., can solve problems such as difficult time-consuming, poor real-time performance, complex biomechanics, etc., and achieve easy modeling and prediction The effect of small force error
- Summary
- Abstract
- Description
- Claims
- Application Information
AI Technical Summary
Problems solved by technology
Method used
Image
Examples
Embodiment Construction
[0022] The present invention will be further described below in conjunction with the accompanying drawings.
[0023] Such as figure 1 As shown, the steps of this embodiment are as follows:
[0024] Step 1: As attached figure 1 As shown in Fig. 1, select a piece of soft tissue sample to be modeled, and place it flat under the mechanical arm with a puncture needle at the end and a force sensor connected to it. Control the robotic arm to puncture the soft tissue. Since the soft tissue is damaged after the puncture, repeated puncture cannot be performed. After each round of puncture, the next puncture point needs to be adjusted to avoid affecting the accuracy of data collection by puncturing at the same point. Ensure that the number of punctures in the final experiment is greater than 5, and enough sample data is collected.
[0025] Step 2: Obtain the position, speed, and acceleration data of the puncture needle through the robotic arm, and obtain the puncture force data throug...
PUM
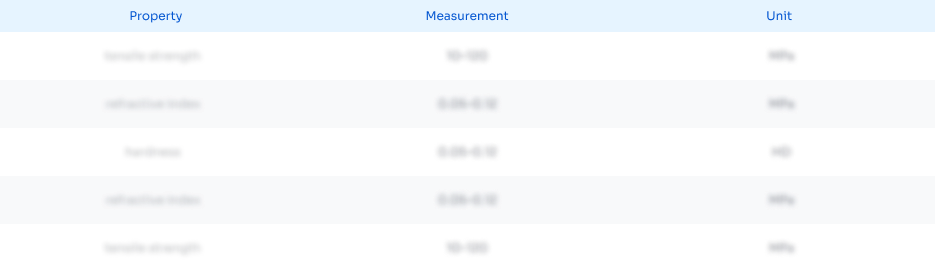
Abstract
Description
Claims
Application Information
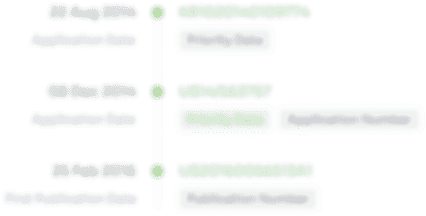
- R&D Engineer
- R&D Manager
- IP Professional
- Industry Leading Data Capabilities
- Powerful AI technology
- Patent DNA Extraction
Browse by: Latest US Patents, China's latest patents, Technical Efficacy Thesaurus, Application Domain, Technology Topic, Popular Technical Reports.
© 2024 PatSnap. All rights reserved.Legal|Privacy policy|Modern Slavery Act Transparency Statement|Sitemap|About US| Contact US: help@patsnap.com